AI Audio Transcription: Evolution and Impact
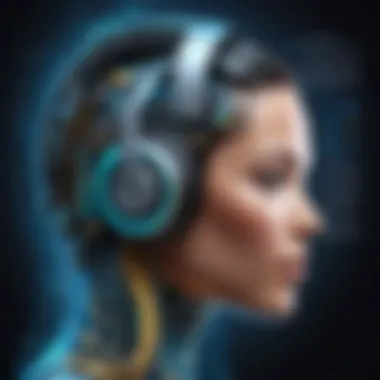
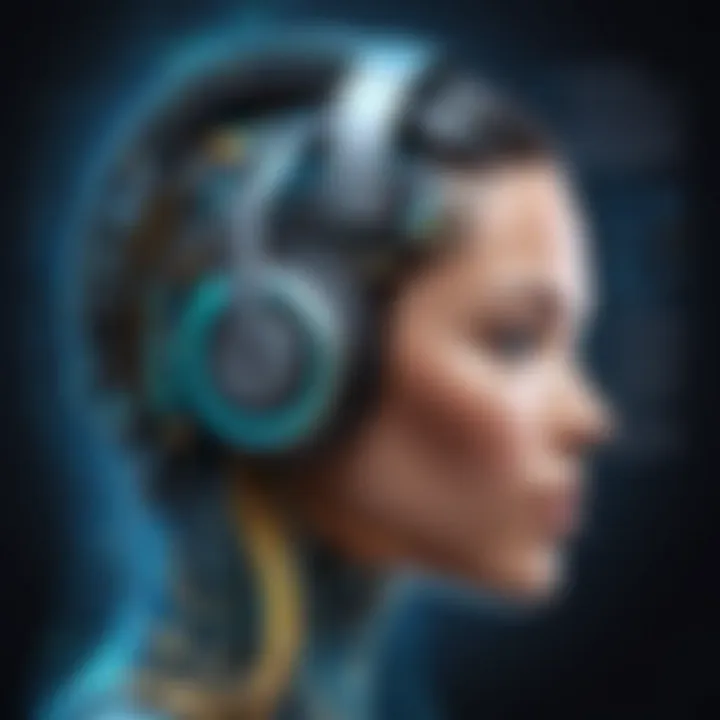
Intro
The rise of artificial intelligence has transformed many sectors. AI audio transcription is one area gaining substantial attention due to its efficiency and effectiveness. Understanding its evolution helps illuminate the implications for various fields like business, education, and content creation. This article reflects on past innovations, existing challenges, and what the future holds for AI audio transcription.
Software Category Overview
Purpose and Importance
AI audio transcription software automates the conversion of spoken language into written text. This technology is essential for streamlining workflows in various industries. It facilitates documentation, enhances accessibility, and improves information retrieval. Many professionals realize the need for efficient transcription, especially in meetings, interviews, or lectures. It saves precious time and resources.
Additionally, accuracy in transcription is crucial. Businesses can avoid misunderstandings and improve communication. Overall, the importance of this software cannot be underestimated, as it serves a fundamental purpose across multiple disciplines.
Current Trends in the Software Category
AI audio transcription continues to evolve. The latest trends include:
- Artificial Intelligence, deep learning, and natural language processing technologies are becoming more refined. This results in greater transcription accuracy.
- Features like speaker identification and punctuation placement are now more commonly integrated, enhancing clarity.
- Integration with other tools such as note-taking apps and project management platforms is facilitated by APIs.
- Focus on multilingual support to accommodate a global workforce.
These trends position AI transcription software as indispensable for modern enterprises.
Data-Driven Analysis
Metrics and Criteria for Evaluation
Evaluating the effectiveness of AI audio transcription tools requires specific metrics. Important considerations include:
- Accuracy Rate: The percentage of correctly transcribed words in comparison to spoken content.
- Speed of Transcription: The time it takes to produce transcripts from audio data. Quick turnaround is crucial for businesses.
- Ease of Use: How simple the interface is for the user. A user-friendly design increases adoption rates.
- Integration Capabilities: Compatibility with existing tools impacts workflow efficiency.
- Cost-Effectiveness: Assessment of value relative to functionality offered.
Comparative Data on Leading Software Solutions
Choosing AI audio transcription software can be daunting. Below are a few leading solutions to consider:
- Otter.ai: Known for its real-time transcription capabilities and user-friendly interface. Great for meetings.
- Rev: Offers both AI and human transcription services. It’s reliable and accurate but higher in cost.
- Sonix: Focuses on speed and multiple language support, making it ideal for international projects.
- Descript: Combines transcription with editing features. It's a preferred choice for content creators.
Given the various needs of users, comparisons are essential to identify which solution suits a particular use case best.
"AI audio transcription is reshaping how we communicate and share knowledge, bridging gaps in accessibility and efficiency."
As technology moves forward, staying informed about these software solutions is vital for making the best choices.
Understanding AI Audio Transcription
AI audio transcription refers to the automatic conversion of spoken language into written text using artificial intelligence technologies. Understanding this field is critical due to its widespread applications and implications across various sectors including business, education, and media. The way we handle and manage audio data has evolved significantly in recent years. As such, being knowledgeable about the definition and historical context of AI audio transcription is essential.
Definition and Key Concepts
At its core, AI audio transcription encompasses several technologies that work synergistically to produce accurate written representations of speech. Speech recognition is the primary component, involving the identification and processing of spoken words through algorithms. Natural language processing (NLP) also plays an integral role by enabling machines to understand and interpret human language. Together, these technologies empower applications to produce transcripts that are not only accurate but contextually relevant.
Most transcription systems utilize machine learning models. These advanced models have been trained on vast datasets, allowing them to learn patterns and nuances in speech. This training is what distinguishes AI audio transcription from traditional manual transcription techniques, as it minimizes human error and enhances overall efficiency.
Historical Context
The journey of AI audio transcription began decades ago, but substantial advancements have occurred more recently. The first speech recognition systems emerged in the 1950s, yet they were limited and only capable of recognizing a small set of words. As computing power improved and exposure to larger datasets increased, the performance of these systems began to evolve.
In the late 20th century, systems such as IBM's ViaVoice and Dragon NaturallySpeaking marked significant progress, allowing users to dictate text more fluently. However, these systems largely required cumbersome training and were often impractical for everyday use.
The advent of deep learning in the 2010s marked a turning point. AI models like Google's WaveNet and OpenAI's various neural networks demonstrated unprecedented ability to understand and transcribe speech accurately and efficiently. This transformation has driven widespread adoption across diverse fields, leading to benefits that were previously unattainable.
Understanding the foundation of AI audio transcription provides deeper insight into its complexities and the rapid progression it has undergone. The potential for transformation in numerous industries is significant, and ongoing research is set to expand its capabilities even further.
How AI Audio Transcription Works
Understanding how AI audio transcription functions is essential for grasping its impact in various fields. This technology bridges communication gaps and enhances productivity, especially in business, media, and accessibility contexts. In this section, we will explore the crucial components at work in AI audio transcription, specifically focusing on the underlying frameworks and methodologies that enable accurate and efficient transcription.
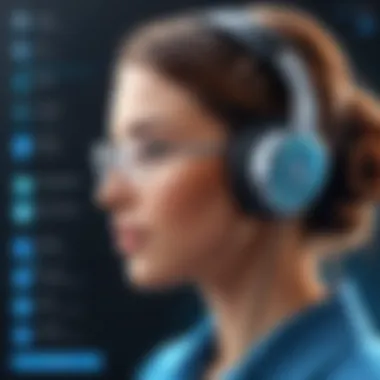
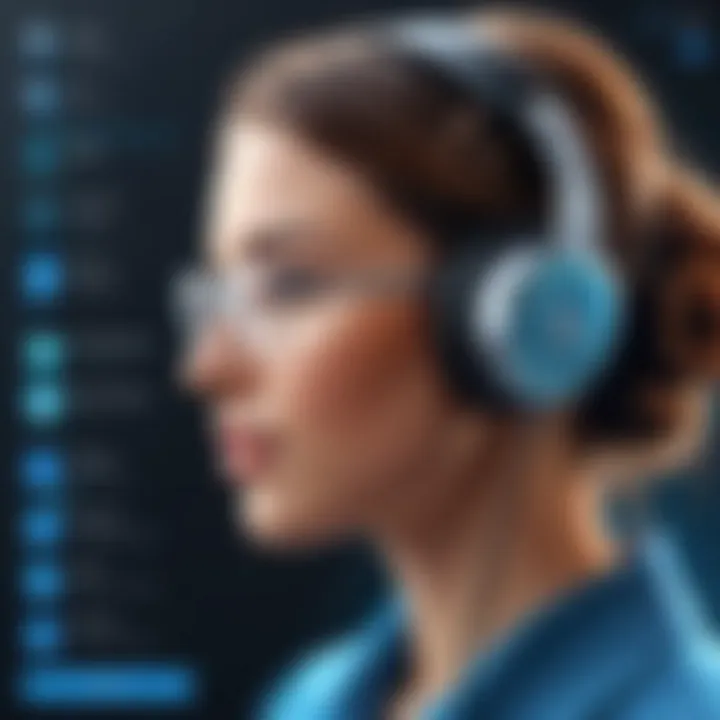
Speech Recognition Algorithms
Speech recognition algorithms form the foundation of AI audio transcription. These algorithms are designed to identify and convert spoken language into written text. They do so through a multi-step process. First, the audio input is divided into manageable segments. Then, features of each segment are analyzed. This may involve breaking sound waves into phonemes, the smallest units of sound. By comparing these sounds to a vast database of known phonemes, the algorithms can accurately interpret speech.
Some key aspects of speech recognition algorithms include their adaptability to various accents and dialects. The more robust the algorithm, the more adept it is at handling different speaking styles. This adaptability is crucial for ensuring broader usability across diverse populations. For example, systems like Google Speech-to-Text and IBM Watson Speech to Text utilize advanced speech recognition algorithms to achieve high accuracy rates.
Natural Language Processing Techniques
Natural Language Processing (NLP) techniques play a significant role in AI audio transcription. While speech recognition converts spoken words to text, NLP ensures that this text is meaningful and contextually accurate. Techniques within NLP include parsing, semantic analysis, and contextual understanding. Parsing breaks down sentences to interpret structure, while semantic analysis assesses the meaning of words within context.
This combination allows AI systems to go beyond mere transcription into understanding nuances such as tone and intent. NLP techniques also enhance transcription accuracy by refining output based on contextual clues. For instance, if a speaker says "bass," the system can determine whether it refers to the fish or a low-frequency sound based on surrounding words.
Machine Learning Models
Machine learning models are integral to improving AI audio transcription over time. These models learn from vast amounts of data. By analyzing previous audio samples and their corresponding transcriptions, the models can identify and correct errors in real-time. Supervised learning is often employed, using datasets that include correct transcriptions as training data.
Additionally, unsupervised learning techniques enable models to further refine their algorithms without explicit instruction. As these models evolve, they become better at recognizing patterns, improving both speed and accuracy. Services like Otter.ai utilize sophisticated machine learning models to enhance transcription quality as they process more audio data.
"AI audio transcription technology is inherently dynamic, learning from each interaction to elevate future performance."
By understanding these components—speech recognition algorithms, natural language processing techniques, and machine learning models—one can appreciate how AI audio transcription not only revolutionizes the act of recording speech but also sets the stage for broader applications in communication and accessibility.
Applications of AI Audio Transcription
AI audio transcription has become a crucial technology across many fields, serving a range of applications that enhance efficiency and accessibility. This section will delve into its significance, exploring how various sectors leverage AI transcription to optimize operations, improve communication, and cater to diverse needs. By understanding these applications, we can appreciate the transformative impact of AI on audio transcription.
Business and Meeting Transcriptions
In the corporate world, timely and accurate documentation is paramount. AI audio transcription facilitates business communication by automatically converting meetings, conferences, and presentations into text format. For example, platforms like Otter.ai and Rev.com provide businesses with the ability to transcribe verbal exchanges in real time.
The benefits are numerous:
- Time-Saving: Managers and teams can focus on discussions rather than taking notes.
- Greater Accuracy: AI models can minimize human error, ensuring that the transcription is accurate.
- Improved Accountability and Record Keeping: Transcriptions provide a clear account of discussions and decisions made during meetings, serving as important documentation.
Media and Entertainment Usage
The media industry is another domain that significantly benefits from AI audio transcription. Content creators and companies like Netflix and Hulu utilize transcription services to produce subtitles and closed captions. This approach not only makes content more accessible but also broadens the audience reach.
Moreover, editors can use transcriptions to quickly analyze footage for editing. This expedites the production process and enhances the overall workflow, making it easier to locate pertinent segments without extensive rewatches.
Academic Research and Study Aids
Academic institutions are leveraging AI audio transcription to assist both students and educators. Transcriptions of lectures provide students with a valuable resource for studying, making it easier to revisit complex topics. Tools such as Google Docs Voice Typing can transcribe spoken language into text, allowing for improved note-taking.
Educators can also transcribe interviews for qualitative research. This aids in coding and analyzing data, promoting a deeper understanding of research subjects. Thus, both students and researchers find AI transcription beneficial for efficiency.
Accessibility for the Hearing Impaired
AI audio transcription plays a pivotal role in enhancing accessibility for individuals who are hearing impaired. By converting spoken language into text, institutions and businesses can ensure that they are catering to a wider audience, providing equal opportunities for learning and participation.
This is highly relevant in educational settings, where captions in classrooms and online courses help remove barriers. Additionally, platforms like YouTube provide autogenerated captions, significantly improving content accessibility for the hearing impaired.
"Transcription technologies offer a bridge to inclusivity for individuals with hearing difficulties, ensuring equal access to information and resources."
Benefits of AI Audio Transcription
AI audio transcription offers numerous advantages that significantly transform how individuals and organizations manage audio data. The importance of this technology is accentuated in its ability to streamline processes, enhance accessibility, and improve overall productivity. Here are some critical elements that underline the benefits of AI audio transcription.
Time Efficiency and Productivity
One of the standout features of AI audio transcription is its ability to save time. Traditional transcription methods can be labor-intensive, often requiring considerable effort from human transcribers. In contrast, AI systems can convert spoken language to written text at a much faster rate.
For instance, AI applications can transcribe hours of audio content within minutes. This capability dramatically reduces turnaround time, allowing professionals to focus on more pressing tasks. The efficiency gained can lead to improved workflow and better utilization of resources. Additionally, businesses that rely on quick documentation of meetings or interviews experience smoother operations with less waiting time.
"Efficiency is not just about doing things faster; it is also about simplifying processes and reducing the time spent on repetitive tasks."
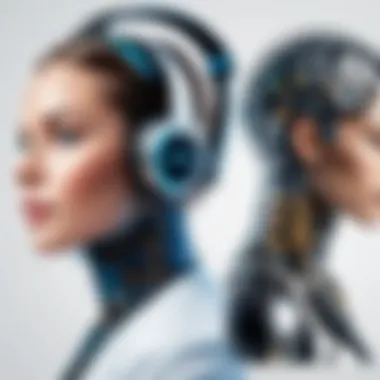
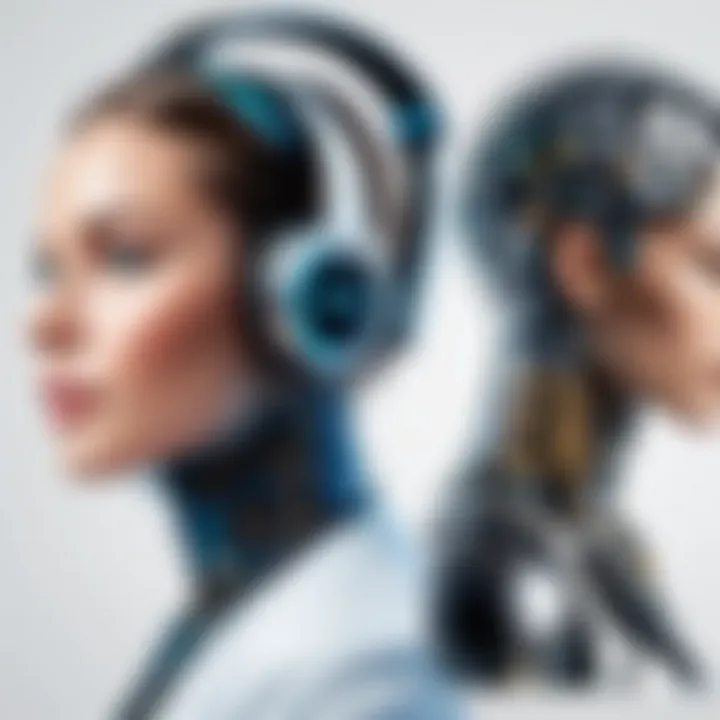
Cost Reduction Compared to Human Transcription
The cost implications of AI audio transcription are noticeable when compared to human transcription services. Employing human transcribers can become expensive, especially when dealing with large volumes of audio material. This is especially true for organizations that need transcription regularly.
While initial investments in AI transcription software might occur, the long-term savings typically outweigh these costs. Organizations can reduce labor expenses and minimize the need for ongoing training and management of human transcribers. Many AI transcription tools also provide flexible subscription models, making it convenient for businesses to scale their usage based on demand.
Enhanced Accuracy with Advanced Algorithms
Accuracy is a critical factor in any transcription service; AI audio transcription continuously improves in this area through advanced algorithms and machine learning techniques. The latest AI models analyze not just the words spoken but also context, punctuation, and even speaker identification. These advancements lead to more precise and coherent transcripts.
Errors in transcription can lead to misunderstandings or loss of critical information. By utilizing AI systems that learn and adapt over time, organizations can achieve a level of accuracy that was challenging to obtain with manual transcription. Businesses can build trust in the reliability of AI-generated transcripts, leading to better decision-making based on accurate information.
Challenges of AI Audio Transcription
In the field of AI audio transcription, various challenges exist that impact the overall effectiveness and usability of these technologies. Understanding these challenges is crucial for not only developers but also end users. Addressing these issues can enhance the performance of transcription systems and improve user experience. The importance of recognizing these challenges lies in their direct implications on accuracy, reliability, and user trust in AI transcription solutions.
Dialect and Accent Variability
One of the foremost challenges in AI audio transcription is the variability of dialects and accents. Different regions have distinct pronunciations, intonations, and even vocabulary. For instance, English spoken in London differs significantly from English in New York or Sydney. Many transcription systems struggle to accurately transcribe speech due to this variability.
This can lead to errors or misunderstandings in the resulting text. Such inaccuracies can be problematic in professional settings where precision is paramount, such as in legal proceedings or medical settings. Moreover, if a transcription tool is primarily trained on a specific accent, it will not perform optimally with others, limiting its usability in diverse environments.
Background Noise and Audio Quality Issues
Background noise poses another significant challenge for AI transcription systems. Various factors contribute to audio quality, including microphone quality, environmental noise, and recording techniques. Transcription algorithms often fail to distinguish between the primary speaker's voice and surrounding sounds. This failure can lead to misinterpretation or omission of crucial information.
Clear audio quality is essential for effective transcription. Users in busy environments, such as conference rooms or coffee shops, may find it difficult to achieve the audio clarity necessary for accurate transcription. Addressing background noise issue often requires sophisticated filtering algorithms, which add to the complexities and computational demands of real-time transcription.
Limitations in Contextual Understanding
Another challenge lies in the limitations of contextual understanding in AI transcription. While advancements in Natural Language Processing (NLP) have improved AI’s ability to understand context, it still struggles with nuances such as idioms, humor, or complex sentence structures. Transcription systems must be capable of contextual interpretation to provide not only accurate transcriptions but also meaningful outputs.
Often, AI models may misinterpret sarcasm or fail to recognize when words are used in a non-literal sense. Such inaccuracies can distort the message being communicated. Furthermore, the lack of contextual awareness can result in repeated errors during transcription, especially in lengthy conversations. Therefore, enhancing contextual understanding is vital for improving the overall effectiveness of AI audio transcription.
Comparison with Traditional Transcription Methods
The shift from traditional transcription methods to AI audio transcription reflects a significant transformation in how information is captured and processed. Traditional transcription typically involves human transcribers who listen to audio recordings and convert them into text. While this method is known for its nuanced understanding of context and tone, it also presents several limitations, especially in terms of time and scalability. With AI audio transcription, we see a new approach that leverages advanced technologies, offering notable advantages.
Accuracy and Speed
One of the foremost advantages of AI audio transcription is its ability to process audio data quickly. AI models, equipped with powerful algorithms, can analyze and transcribe long recordings in a fraction of the time it would take a human. Studies indicate that AI systems can achieve real-time transcription for many applications, which is a notable improvement over the typical turnaround time associated with human transcribers. This speed not only benefits organizations that rely on rapid access to information but also enhances the overall workflow.
However, the accuracy of AI audio transcription can vary. While advancements have led to impressive performance in controlled environments, challenges still arise in understanding varied accents and dialects. Human transcribers may better manage context and background noise, leading to a more accurate representation of the spoken word in certain situations. Companies need to balance the benefits of rapid transcription with the necessity of accuracy, particularly in high-stakes fields such as law or healthcare.
Cost Implications
Cost efficiency is another critical consideration when comparing AI audio transcription to traditional methods. Employing skilled human transcribers can be expensive, especially for projects requiring transcription of hours of audio. In contrast, AI transcription services often operate on a subscription or usage-based model, which can result in significant savings for businesses.
Organizations can save not only on the hourly wages of transcribers but also on the overhead costs associated with hiring and maintaining a transcription team. With AI solutions, the financial implications are especially favorable for businesses that require frequent and extensive transcription services. The initial investment in AI technology may seem substantial, but the long-term cost savings can be considerable.
"The future of transcription is here, reshaping workflows at a pace never seen before."
User Experiences and Testimonials
User experiences and testimonials are essential components in understanding the practical implications of AI audio transcription technologies. They provide real-world insights that can guide potential users in their decision-making process. This section will analyze how professionals interact with AI transcription tools, detailing both the advantages and challenges they encounter. By examining these experiences, readers can gain a clearer perspective on what to expect from such technologies in their workflow.
Personal Accounts from Professionals
Many professionals have shared their experiences using AI audio transcription tools, shedding light on their effectiveness in various sectors. For instance, a market researcher might describe how using tools like Otter.ai has streamlined the process of capturing qualitative data from interviews. The ability to quickly translate spoken words into written form allows researchers to focus more on data analysis rather than on manual note-taking.
"With AI transcription, I can analyze data faster and enhance the quality of my reports. It has changed how I work daily."
In another example, an attorney highlights the benefits of using Trint to manage recorded depositions. The ability to integrate transcription with case management systems ensures that critical information is easily accessible, thus improving case preparation efficiency.
These firsthand accounts illustrate that while satisfaction levels are generally high, they are not uniform across all users. Factors such as the accuracy of transcriptions and the ability to adapt to different dialects or accents can greatly influence user experience.
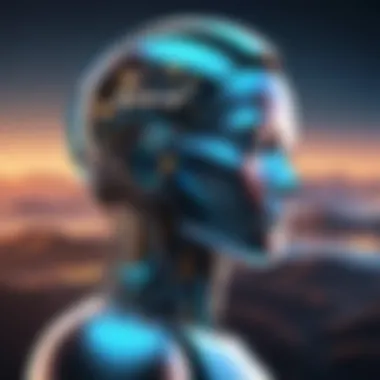
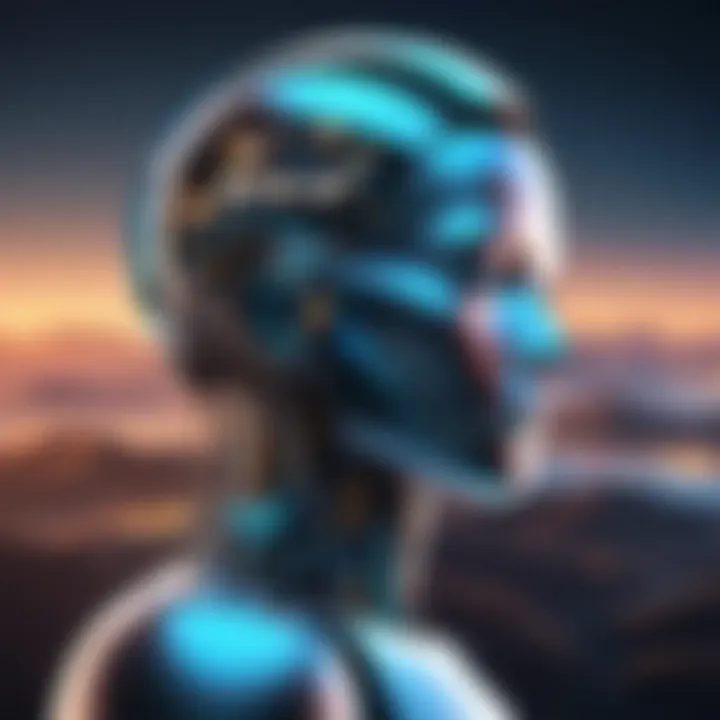
Impact on Workflow and Efficiency
AI audio transcription tools can bring significant improvements to workflow efficiency across multiple professions. Below are some key areas where transcription technology impacts daily operations:
- Time Savings: Professionals report that AI transcription saves hours previously spent on manual transcription tasks. The speed at which recordings are converted into text allows employees to allocate more time toward value-added tasks.
- Accessibility of Information: With transcriptions that are searchable and easy to archive, retrieval of past meetings, interviews, or discussions becomes much easier. This enhances knowledge sharing within teams and organizations.
- Enhanced Collaboration: Teams can instantly share transcription outcomes, making discussions more productive and focused. This shared resource allows for better preparation and follow-up on discussions.
Despite these advantages, it is important to recognize potential drawbacks. In some cases, individuals might find that automated transcriptions still require manual editing for accuracy. This necessity can add an unexpected new layer of work, particularly when high precision is critical.
Understanding the user experience through these testimonials illustrates the dual-edged nature of AI transcription. While the benefits are pronounced, individuals need to be aware of its limitations. The narrative formed by user experiences is not just anecdotal; it carries substantial weight in shaping perceptions of AI transcription technologies.
Future Trends in AI Audio Transcription
The domain of AI audio transcription is on the verge of substantial transformations that promise to reshape its impact across various industries. As technological innovations continue to emerge, it is essential to examine not only the advancements themselves but also their implications for businesses and individuals alike. Here, we will focus on significant developments, potential benefits, and critical considerations surrounding the future of AI audio transcription.
Advancements in Technology
Recent developments in AI have led to powerful improvements in transcription accuracy and efficiency. The advent of deep learning models has brought about a heightened capability for handling large vocabularies while understanding context better. Technologies like Google’s Speech-to-Text and IBM Watson Speech to Text play vital roles in these advancements.
- Improved Natural Language Processing: Natural Language Processing is becoming increasingly sophisticated. This allows for better comprehension of nuances in human speech, such as idioms, jargon, and even emotional undertones.
- Real-time Processing: Users can expect real-time transcription to become more common. This means that as discussions occur, transcription will keep pace without lag, drastically enhancing the utility of these technologies during live events.
- Multi-language Support: The globalized world demands that transcription services cater to diverse languages and dialects, which many emerging technologies are now facilitating more effectively. Companies are working towards systems that can dynamically switch between languages based on an individual speaker's preference.
"The future of AI audio transcription technology promises to transcend traditional boundaries and deliver an experience tailored to user needs."
Integrations with Other Technologies
Integrating AI transcription with other technologies can optimize workflows and enhance user experiences significantly. Here are some critical facets of these integrations:
- Collaboration Tools: Incorporating transcription services into tools like Slack, Microsoft Teams, and Zoom can streamline communication. Organizations increasingly rely on seamless connectivity, allowing teams to focus on work without worrying about minute details.
- Voice Assistants: The synergy between audio transcription and voice-enabled AI, such as Google Assistant or Amazon’s Alexa, presents an engaging area for growth. Enhanced transcription accuracy is vital for these systems to function efficiently in various environments.
- Cloud Storage: Transcription can also be integrated with cloud services like Google Drive or Dropbox. This allows users to access their transcribed data effortlessly, ensuring that important discussions, meetings, and lectures are archived efficiently.
As these integrations develop, the landscape of AI audio transcription is poised to become an integral part of a vast digital ecosystem, enhancing productivity and communication across sectors.
Ethical Considerations
The utilization of AI audio transcription technology raises significant ethical questions that cannot be ignored. These considerations affect not only the design and deployment of these tools but also the perceptions and rights of users involved. With the evolution of this technology, it is vital to assess the implications that stem from its integration into various fields.
Privacy and Data Security
When individuals use AI audio transcription services, they often share sensitive information. This information can be personal conversations, business meetings, or confidential interviews. Protecting this data is crucial. Companies must implement robust data security mechanisms to safeguard user information.
Moreover, users must be clear about how their data will be used. For instance, will it be stored, shared with third parties, or utilized for training AI models? Transparency plays a vital role in establishing trust between users and service providers.
- Best practices for ensuring privacy include:
- Implementing end-to-end encryption
- Limiting data retention periods
- Regular audits of data security practices
These strategies can enhance the assurance of users regarding their data privacy.
Bias and Fairness in AI Models
The concept of bias in AI audio transcription is another pressing concern. AI models are trained on vast datasets, which may include inherent biases. As a result, they may perform poorly with certain dialects, accents, or demographic groups. This can lead to unfair treatment and exclusion in areas where accurate transcription is critical.
Addressing bias requires a multifaceted approach. Developers need to curate diverse datasets that reflect a broad range of voices and dialects. Additionally, continuous testing and refinement of the models must occur to ensure they respond equitably across different user groups.
Some strategies to reduce bias may include:
- Diverse training datasets: Ensuring comprehensive representation of various languages and accents.
- Regular evaluation: Routinely assessing AI performance across demographic variations.
- User feedback loops: Encouraging users to report inaccuracies, which in turn helps improve model performance.
"Ethical AI isn't just about technology. It involves understanding and minimizing the impact of biases present in the models."
The responsibility lies with developers and stakeholders to promote an equitable application of AI audio transcription tools while keeping user privacy a priority.
End
Summary of Key Insights
The advancements in AI audio transcription stem from a combination of sophisticated speech recognition algorithms and natural language processing techniques. They have enhanced efficiency by providing near-instantaneous transcriptions, thus allowing users to focus on higher-level tasks. The applications cover a wide spectrum, impacting business, entertainment, academia, and accessibility for those with hearing impairments. Importantly, we noted the balance of benefits, including time savings and cost efficiency, against challenges such as dialect variability and background noise.
"AI audio transcription opens new doors for communication and efficiency, but understanding its effectiveness demands a clear perspective on its current capabilities and limitations."
Implications for Future Research and Development
As AI audio transcription technology continues to evolve, several implications arise. Future research should focus on improving the contextual understanding of AI models, addressing biases, and enhancing data security measures. Such advancements can lead to more robust and equitable systems, ultimately benefiting all users. Integrating audio transcription with emerging technologies like machine learning and artificial intelligence can unlock new capabilities and further refine the accuracy and reliability of these tools.