Top Data Transformation Tools for 2023
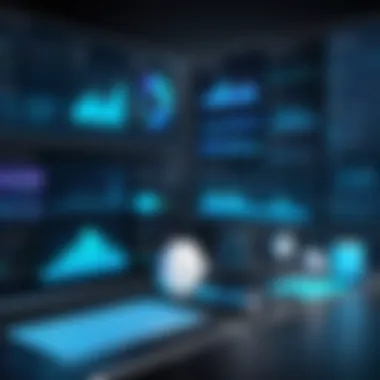
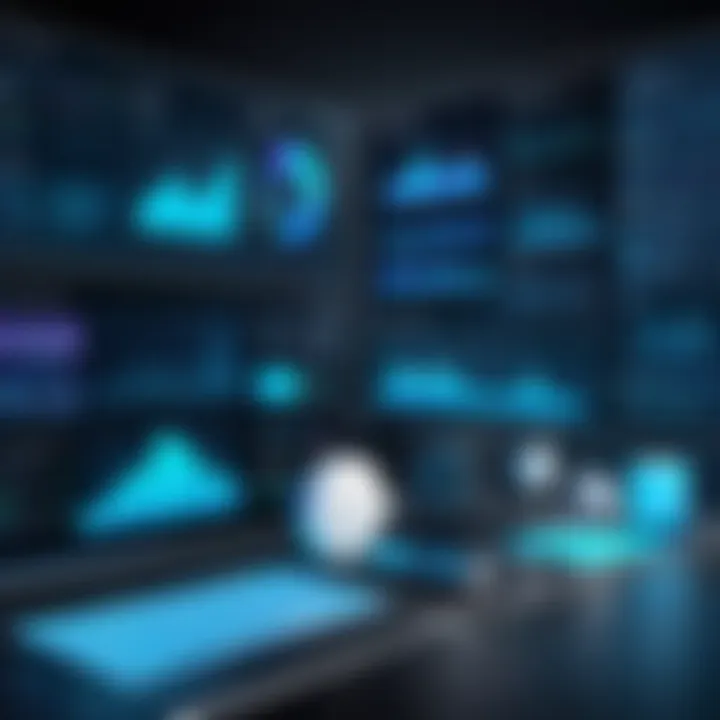
Intro
Data transformation tools form an essential part of the data management ecosystem. They allow businesses and organizations to convert data from one format or structure to another, making it usable for various applications. In an age where data-driven insights are vital to decision-making, selecting the right transformation tool can significantly impact efficiency and outcome. This article aims to explore the leading data transformation tools by discussing their key features, use cases, and integration capabilities.
Software Category Overview
Purpose and Importance
Data transformation tools serve to facilitate the seamless flow of information across different systems. They enable organizations to integrate, transform, and optimize their data for analysis and reporting. In a landscape marked by vast amounts of data, these tools ensure that information is accessible, relevant, and actionable. Effective data transformation can improve data quality, reduce redundancy, and enhance overall data governance.
Current Trends in the Software Category
In recent years, there has been a notable shift towards cloud-based data transformation solutions. Organizations are increasingly adopting platforms such as AWS Glue, Microsoft Azure Data Factory, and Google Cloud Dataflow. These tools offer scalability, flexibility, and ease of integration with other cloud services. Additionally, there is a growing emphasis on automation in data transformation processes. By employing machine learning algorithms, these tools can streamline workflows and improve accuracy.
Data-Driven Analysis
Metrics and Criteria for Evaluation
When assessing data transformation tools, it is important to consider several key metrics:
- Ease of use: User-friendly interfaces promote quicker adoption.
- Integration capabilities: Ability to connect with various data sources.
- Performance: Processing speed can affect timely insights.
- Scalability: Can the tool adapt to increasing data volumes?
- Cost-effectiveness: Total cost of ownership including licensing and operational costs.
Comparative Data on Leading Software Solutions
In the current market, a small number of tools stand out due to their robust capabilities. Tools like Talend, Informatica PowerCenter, and Apache NiFi have established a strong reputation among professionals. Each offers unique functionalities tailored for specific use cases.
"Choosing the right data transformation tool is crucial for optimizing data flow and gaining actionable insights."
Talend provides an open-source platform that emphasizes collaboration and data quality. It is ideal for organizations wanting customizable solutions.
Informatica PowerCenter stands as a leader in enterprise data integration, famed for its comprehensive functionality that meets the demands of large organizations.
Apache NiFi offers a flexible architecture for automating data flows, useful for dynamic and fast-changing data environments.
As businesses look for solutions to enhance their data management practices, understanding these tools and their applications will serve as a crucial step in fostering informed decisions.
Foreword to Data Transformation
In today's data-driven landscape, the process of data transformation holds significant importance. It involves converting data from one format or structure to another, ensuring that it is suitable for analysis and further action. This transforms raw data into insightful information, driving better decision-making across various sectors. As data continues to grow in volume and variety, the ability to efficiently transform data is crucial for organizations aiming to leverage analytics for competitive advantage.
Definition of Data Transformation
Data transformation is a systematic process where data is converted into a format that is more suitable for analysis, reporting, or other types of operations. This can include changing the structure, format, or values of data. It involves various techniques, such as merging, aggregating, and cleaning data to improve its quality. Typical transformation processes can range from extracting data from disparate sources to reformatting it to fit a specific schema. This definition encapsulates the essence of data transformation — making data useful and actionable.
Importance in Data Management
The role of data transformation is paramount in effective data management. Without proper transformation, organizations risk dealing with inaccurate or inconsistent data, which can lead to severe consequences. The benefits of effective data transformation include:
- Data Quality Improvement: Ensures that data is clean, accurate, and reliable for decision-making.
- Enhanced Analytics: Facilitates better visualization and analysis, enabling deeper insights.
- Streamlined Processes: Automates data handling, minimizing manual errors and improving efficiency.
- Seamless Integration: Allows for better data integration across different systems and platforms, enhancing collaboration.
In summary, data transformation is not just a technical requirement; it is a vital process that impacts an organization’s ability to harness the power of data. By prioritizing effective transformation practices, businesses can optimize their data management strategies and drive more informed decisions.
Criteria for Evaluating Data Transformation Tools
Selecting the right data transformation tool is critical for any organization. Each tool has its own set of features, strengths, and weaknesses. Therefore, understanding the criteria for evaluating these tools lays a strong foundation for informed decision-making. This section outlines several key factors that every professional should consider when assessing data transformation solutions.
Functionality
Functionality is the core aspect of any data transformation tool. It defines what a tool can do. A capable tool should perform operations like data cleansing, data integration, transformation, and aggregation seamlessly. Users must examine specific features that meet their data needs.
For example, some tools may have advanced features for data manipulation and conversion while others might offer basic capabilities. Evaluate how the tool handles diverse data formats, as well as its ability to automate repetitive tasks. Look for user-defined functions that enhance customization. A tool’s ability to scale its functionality based on demand can save both time and resources.
Ease of Use
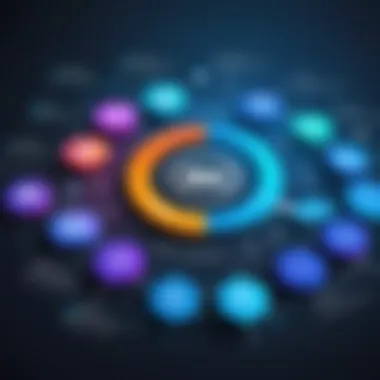
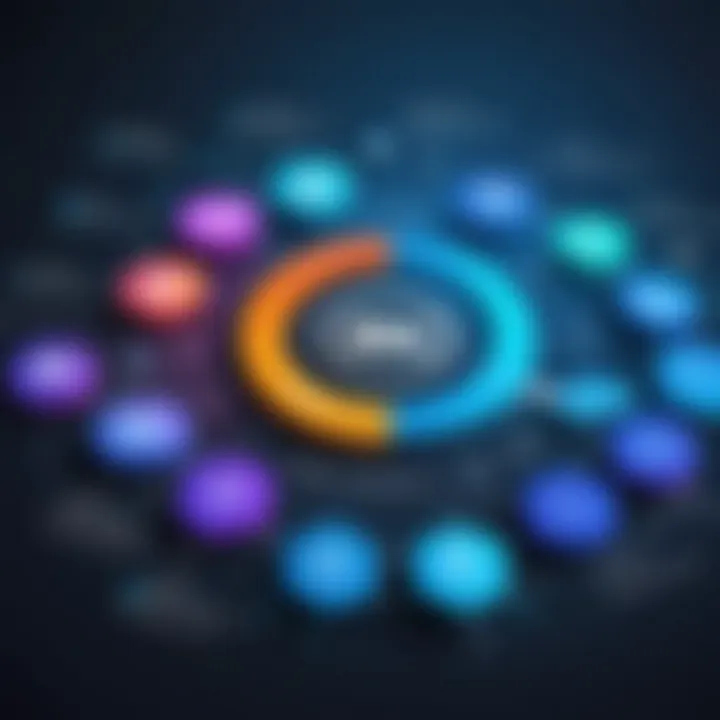
A tool can have advanced functionality, but if it is not user-friendly, its potential remains untapped. Ease of use is about the user experience. A good tool should have a straightforward interface and intuitive navigation. Users should find it easy to manipulate data without extensive training.
Initial setup and configuration should be smooth. Having a well-organized dashboard simplifies monitoring tasks like data flow and quality assurance. Look for documentation and support resources that assist users in overcoming any challenges. Ultimately, time spent learning can impact productivity levels.
Integration Capabilities
In today’s data landscape, integration cannot be overlooked. Tools must work well with other systems to create a streamlined workflow. Seek tools that integrate with databases, cloud services, and a variety of applications. Strong integration capabilities allow for real-time data access.
Compatibility with APIs and existing data lakes is essential. This means professionals can transfer data between systems efficiently. A lack of integration can create silos and hinder data visibility. Therefore, tools that facilitate connection across platforms help in making data transformation more effective.
Scalability
As organizations grow, so do their data needs. Scalability refers to a tool’s capacity to handle an increasing volume and complexity of data without losing performance. A scalable tool should adapt to the ongoing changes in data requirements over time.
Whether adding new data sources or expanding user access, scalability is vital. Evaluate how the tool handles peak loads and whether it can be optimized for greater efficiency when needed. Organizations should not find themselves constrained by their tools as they evolve.
Cost Considerations
Cost is often a deciding factor in selecting a data transformation tool. Businesses must assess total ownership costs, including licensing, maintenance, and support fees. Look for transparent pricing models that fit within budget constraints.
Some tools may offer a free version with limited features, which can help organizations test the solution before committing. Weigh the costs against the potential efficiency gains and time savings the tool can provide. Sometimes, investing more upfront can lead to long-term benefits worth the expense.
Evaluating these criteria helps organizations choose a tool that not only meets current needs but also aligns with future data strategies.
Overview of Leading Data Transformation Tools
In the expansive field of data management, the role of data transformation tools is crucial. They serve as the backbone for converting raw data into usable insights, which significantly enhances decision-making for businesses. There are several tools today that provide various functionalities tailored to specific data transformation needs. Understanding these tools can lead to smarter data handling practices.
The selection of a suitable data transformation tool can make a considerable difference in efficiency and effectiveness. This section will carefully analyze five prominent tools in the market. Each tool has its unique set of features that can align with different user requirements. It is essential to evaluate these tools not just based on price but also on capability, performance, and support.
Tool A: Features and Benefits
Tool A stands as a powerhouse in data transformation. It allows seamless data extraction from multiple sources and provides reliable integration. Its key features include:
- User-Friendly Interface: The tool is designed for easy navigation, which reduces the learning curve for new users.
- High-Speed Processing: With advanced algorithms, Tool A can handle large datasets swiftly, ensuring that data flows efficiently.
- Customizable Workflows: Users can design workflows tailored to meet specific project demands.
The benefits of using Tool A are notable. It minimizes errors during the data transformation process and enhances overall productivity, making it ideal for businesses that require quick and reliable data processing.
Tool B: Features and Benefits
Tool B has gained popularity due to its robust integration capabilities. This tool excels in connecting various platforms, making data migration a seamless experience. Important features include:
- Robust API: Data can be pulled from various sources effortlessly.
- Data Quality Monitoring: It regularly checks data integrity to ensure accuracy.
- Real-Time Processing: Tool B is capable of processing data in real-time, which is beneficial for businesses needing up-to-date information.
Users of Tool B often report improved collaboration across teams. Its strengths in integration allow for more cohesive data strategies, making it a favored choice for companies handling diverse datasets.
Tool C: Features and Benefits
Tool C brings an intuitive approach to data transformation. It focuses on automating repetitive tasks, reducing manual intervention. Here are its key features:
- Automated Scripts: These reduce the workload on users.
- Visual Data Mapping: Tool C offers visual representations which assist in monitoring data flows and transformations better.
- Comprehensive Reporting: Regular reports help in tracking performance and spotting issues early.
The automation features in Tool C significantly decrease the risk of human error. Additionally, its reporting functionalities give users insight into their data practices, enabling better decision-making.
Tool D: Features and Benefits
Tool D stands out with its emphasis on security and compliance. For businesses managing sensitive data, this tool ensures that all transformations adhere to industry standards. Key features encompass:
- Encryption Protocols: Data is encrypted both in transit and at rest.
- Compliance Reporting: Assisted reporting features ensure that users can easily demonstrate compliance with regulations.
- User Access Controls: Administrators can manage permissions to enhance data security.
Given its strong security measures, Tool D is suitable for sectors such as finance and healthcare where data privacy is paramount.
Tool E: Features and Benefits
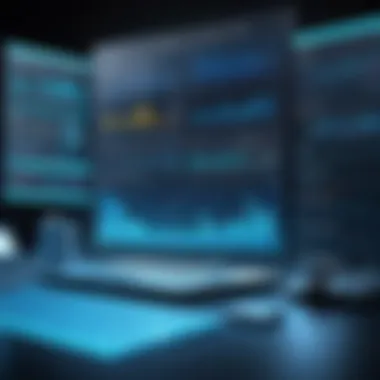
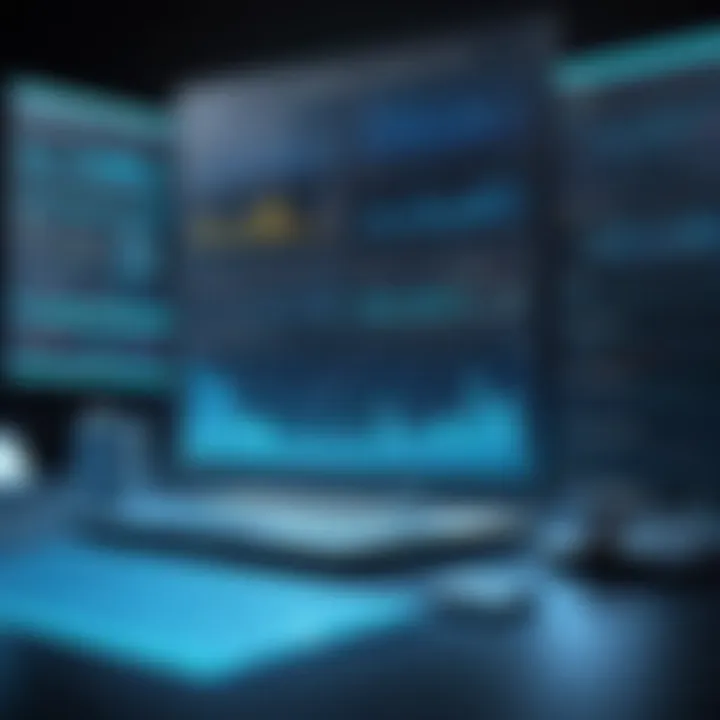
Lastly, Tool E is lauded for its cost-effectiveness. It offers many of the same functionalities as its competitors but at a more accessible price. Essential features include:
- Flexible Pricing Tiers: Catering to small businesses and large enterprises.
- User Community: A vibrant user forum provides support and best practice sharing.
- Integration Templates: Pre-built templates facilitate faster setup with common applications.
The affordability of Tool E makes it enticing for startups and small businesses looking to optimize their data processes without the fear of overspending.
The choice of a data transformation tool has long-term implications for an organization’s data strategy. It is vital to consider not just immediate needs but future scalability as well.
Comparative Analysis of Tools
Comparative analysis of data transformation tools is crucial in understanding their strengths and weaknesses. This analysis provides a structured approach to evaluate the platforms available in the market based on key performance metrics. By examining specific elements such as speed, data quality, user experience, and costs, professionals can make informed decisions. The significance lies in its ability to reveal how each tool aligns with the unique needs of an organization, ensuring the optimal choice for data management goals.
Performance Metrics
Speed of Transformation
Speed of transformation is a key aspect that directly impacts workflow efficiency. A tool's ability to rapidly process and convert data can significantly shorten project timelines. Choosing a tool with high transformation speed contributes to improved productivity and quicker access to actionable insights. This becomes especially crucial for organizations that rely on real-time decision-making. Therefore, tools like Talend and Apache Nifi are recognized for their fast processing capabilities, allowing businesses to stay agile in dynamic market conditions.
Data Quality Assurance
Data quality assurance stands as a foundational element in data transformation. Maintaining high-quality data reduces errors and enhances decision-making accuracy. Tools that emphasize data validation and cleansing are essential in ensuring that the data meets the required standards. The prominent characteristic of data quality assurance in leading tools is the implementation of automatic checks. These checks help identify inconsistencies and correctness of data before it is transformed. This feature is beneficial in maintaining a reliable data pipeline, ensuring that stakeholders always work with accurate information.
User Experience
User Feedback and Reviews
User feedback and reviews provide insights into the practical experience of using a data transformation tool. By analyzing reviews, businesses can gauge the satisfaction levels of current users and understand potential challenges. This perspective is valuable for evaluating the usability and effectiveness of tools. Strong user feedback often points towards intuitive interfaces and robust customer support, making it a crucial consideration. Tools like Alteryx have received positive reviews related to user-friendliness, which enhances overall satisfaction.
Interface Design
Interface design plays a vital role in user experience. A well-designed interface facilitates easier navigation and enhances the productivity of users. The characteristic of a good interface includes clarity and accessibility of features which can drastically improve the learning curve. In the context of data transformation, an intuitive interface reduces the time users spend figuring out the system and allows them to focus on the actual data handling. Therefore, tools with effective interface design are often favored in various business environments as they encourage user engagement.
Cost Analysis
Pricing Models
Pricing models are fundamental when evaluating data transformation tools. Different tools offer various pricing structures, impacting total cost considerations for companies. Flexible models, such as subscription-based or tiered pricing, allow organizations to choose options that match their budget and usage needs. A popular choice for many businesses is the subscription model. This approach can often lower initial costs while providing access to continuous updates and improvements.
Total Cost of Ownership
The total cost of ownership (TCO) includes all costs associated with a data transformation tool over its lifespan. This encompasses initial purchase price, maintenance, and any additional training or support costs. Understanding TCO is vital for businesses as it goes beyond simple upfront costs. A thorough analysis reveals what the real investment is over time and helps ensure that financial resources are allocated effectively. Tools with high TCO might be less appealing if accessible alternatives can deliver comparable results at a lower long-term expense.
"Selecting the right data transformation tool is not solely about initial costs, but rather the full implications of ownership and value it brings to your data management strategy."
By conducting a comparative analysis of these aspects, organizations can substantively enhance their data management processes. Identifying effective tools leads to streamlined operations and improved data integrity.
Common Use Cases for Data Transformation Tools
Data transformation tools play a crucial role in various sectors, facilitating the manipulation and conversion of data into a usable format. Understanding these common use cases is essential for organizations looking to enhance their data processes. The significance lies not just in the efficiency of data handling, but also in the improved decision-making and insights that result from effective data management.
Data Migration Projects
Data migration involves transferring data between storage types, formats, or systems. It's a critical task when organizations upgrade systems or consolidate data. Choosing the right data transformation tool can minimize downtime and improve accuracy during this process.
For example, the migration of customer data from a legacy system to a cloud-based solution needs careful mapping of data fields. Tools like Talend or Informatica can automate many aspects of this process, ensuring all necessary data is transferred with integrity. Additionally, they often provide validation features that help verify the migration results. Thus, leveraging such tools can improve efficiency and reduce the risk of errors.
ETL Processes
Extract, Transform, Load (ETL) processes are fundamental in data analytics. They involve extracting data from various sources, transforming it into a consistent format, and loading it into a data warehouse. ETL is essential for enabling organizations to analyze data more effectively.
Using transformation tools in ETL processes helps maintain data quality and consistency. For instance, Apache Nifi and Microsoft SQL Server Integration Services (SSIS) offer robust ETL features. They allow users to automate data preparation, which saves time and resources. Moreover, the ability to integrate with different databases means organizations can work with diverse data sources seamlessly. This streamlined process is key to delivering timely and relevant insights.
Data Quality Improvement
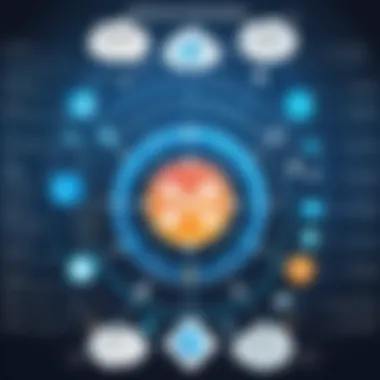
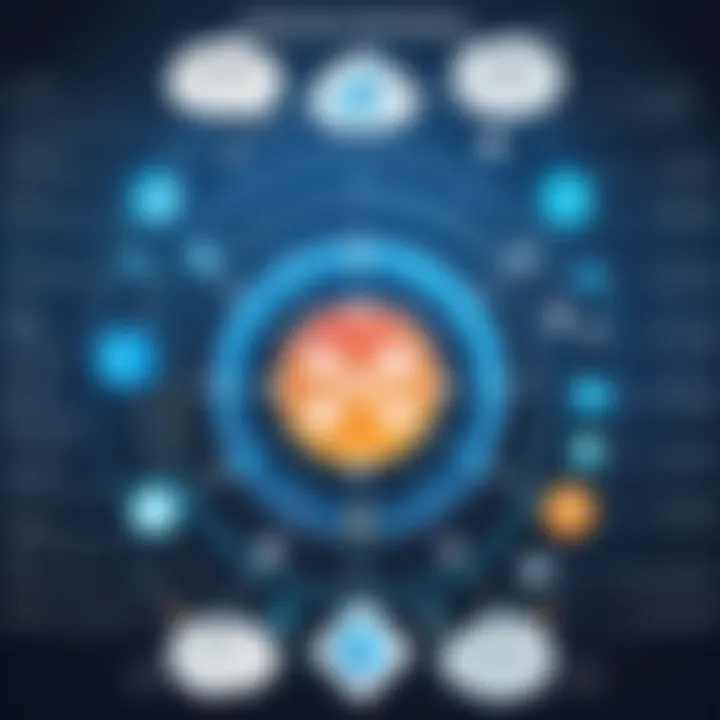
Data quality is vital for any organization since errors can lead to poor decision-making. Transformation tools can aid in cleaning and enriching datasets to enhance overall data quality. This includes identifying duplicates, correcting inconsistencies, and filling gaps in data.
Tools like data profiling features in Python libraries can help assess the quality of datasets before they are used in analysis. Such tools provide detailed metrics and reports, which allow data professionals to target specific areas of improvement. Ensuring high data quality not only boosts trust in data-driven decisions but also enhances operational efficiency.
Real-time Data Processing
In an age where immediate data analysis is critical, real-time data processing cannot be overlooked. Many organizations require up-to-the-minute data insights for various operations, including marketing, sales, and customer support. Transformation tools can facilitate near real-time processing by allowing data to be transformed and analyzed as it enters the system.
For example, tools like Apache Kafka or Amazon Kinesis can handle large streams of data effectively. These tools enable organizations to process data in motion, which offers the ability to react quickly to changing conditions. Real-time processing allows businesses to stay ahead of the competition by adapting strategies based on the latest data.
'Efficient data transformation is not just a technical need; it’s a strategic advantage for organizations.'
By understanding these common use cases, organizations can better select the right tools to suit their needs. Focusing on data migration, ETL processes, data quality improvement, and real-time processing helps align data strategies with business objectives.
Future Trends in Data Transformation
The landscape of data transformation is evolving rapidly as technology advances and organizations strive for improved efficiencies. Understanding future trends in data transformation is crucial, as they can significantly influence the way businesses handle their data. With the rise of automation, AI, and more secure practices, keeping abreast of these trends can provide a competitive edge.
Automation and AI Integration
Automation is becoming a pivotal aspect of data transformation. Organizations are looking for ways to minimize manual processes while maximizing accuracy and speed. The integration of Artificial Intelligence in data transformation tools enhances the capability to process large datasets quickly.
Benefits of incorporating automation and AI include:
- Efficiency: Automated tools can handle routine tasks, saving time for more strategic work.
- Error Reduction: AI can analyze patterns and learn from past mistakes, leading to fewer data quality issues.
- Scalability: AI systems can easily adapt to changing data requirements.
Organizations that embrace these technologies are likely to see not just improvements in productivity but also a significant return on investment.
Enhanced Security Features
As data breaches become increasingly common, security is paramount in data transformation. Future tools are expected to incorporate even more robust security features.
Considerations for enhanced security include:
- Data Encryption: Ensuring that sensitive information is encrypted during transformation processes.
- Access Controls: Implementing strict user authentication protocols to protect data integrity.
- Compliance: Monitoring for compliance with regulations like GDPR and CCPA during data handling.
Security in data transformation tools will be essential for fostering trust among users and protecting organizational data from external threats.
Open Source Solutions
Open-source tools in data transformation are gaining popularity. Such solutions provide flexibility and customization, allowing businesses to tailor functionalities to their needs.
Key advantages of open source tools are:
- Cost-Effective: Many open-source solutions are free or low cost, reducing overall expenses.
- Community Support: Users can benefit from the knowledge and experience of a broad user community.
- Transparency: Open-source software allows organizations to scrutinize code, helping them identify potential vulnerabilities or inefficiencies early in the process.
Adopting open-source tools can ultimately lead to more innovative and secure data transformation practices.
"In the fast-paced world of data management, staying ahead of trends ensures that organizations can leverage data effectively while mitigating risks."
Closure
Data transformation is crucial in today's dynamic data landscape. This article has explored various aspects of data transformation tools, highlighting their impact on data management. Selecting the right tool becomes an essential task for organizations aiming to leverage data effectively. The tools discussed offer various features, enabling better handling and presentation of data.
Summary of Key Points
In this article, several important points were discussed regarding data transformation tools:
- Functionality and Features: Each tool presents unique capabilities tailored to different business requirements. From ETL processes to real-time data processing, understanding these features is vital.
- Use Cases: Knowing specific applications of these tools helps users identify what suits their data needs better. Data migration, quality improvement, and integration with existing systems were examined.
- Market Trends: The future must consider automation, AI integration, and security enhancements. Tools that adapt to these trends will likely perform better as data needs evolve.
"Choosing the right data transformation tool can create significant efficiencies within data management processes."
Recommendations for Selection
When selecting a data transformation tool, consider the following:
- Functionality: Evaluate the specific features necessary for your business objectives. Are you focused on data migration, quality enhancement, or real-time processing?
- Integration: Ensure that the tool can seamlessly integrate with your existing systems. Compatibility is key for a smooth transition and operation.
- Scalability: Choose a tool that can grow with your business needs. Consider current and future demands to prevent costly changes down the line.
- Cost: Analyze total cost of ownership, including licensing, support, and potential training needs.
By carefully assessing these elements, organizations can select data transformation tools that enhance their data management strategies effectively.