Top Data Warehouse Solutions for Modern Enterprises
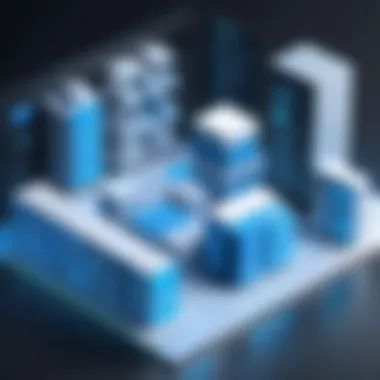
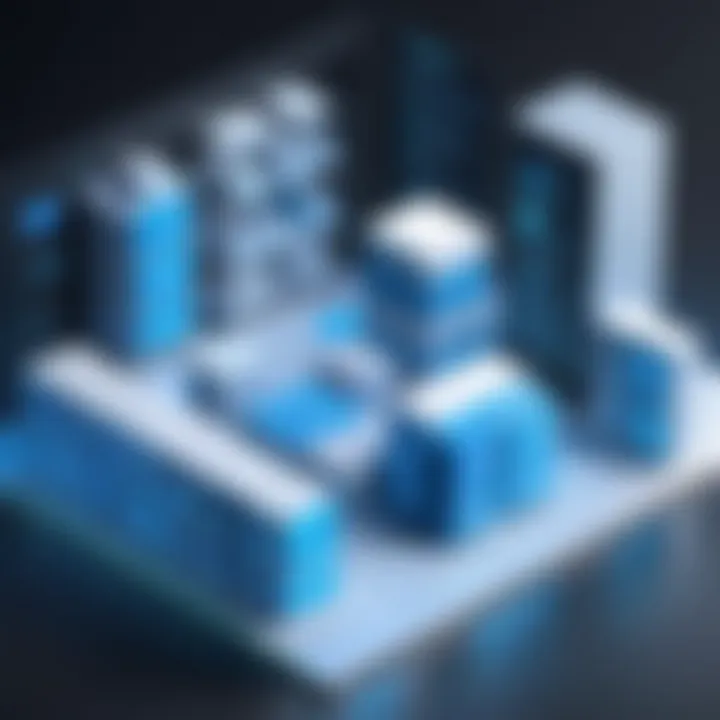
Intro
In today's data-driven world, the establishment of a robust data warehouse is critical for modern enterprises. As organizations increasingly rely on data to drive decision-making, the need for efficient, scalable, and cost-effective data storage solutions becomes paramount. McKinsey and Company states that data-driven companies are 23 times more likely to acquire customers and 6 times more likely to retain them. Therefore, understanding optimal data warehouse solutions is not just a luxury; it is a necessity.
This article delves into several key elements that define the landscape of data warehousing today. It addresses performance, scalability, and cost-effectiveness while providing comparisons among leading platforms. As enterprises navigate through numerous available options, having a clear framework for evaluation becomes essential. The insights presented in this comprehensive guide will assist decision-makers in selecting a solution that aligns with their unique organizational needs.
Software Category Overview
Purpose and Importance
A data warehouse serves as a centralized repository for an organization’s data. It allows for efficient storage, processing, and retrieval of data from various sources. The primary purpose is to optimize data analysis and reporting, leading to better informed business strategies. An effective data warehouse solution can significantly enhance an enterprise's ability to manage vast amounts of data, facilitating advanced analytics, business intelligence, and reporting.
Current Trends in the Software Category
The realm of data warehousing is not static. Trends such as cloud computing, artificial intelligence, and real-time analytics are shaping how organizations implement their data strategies. Here are some of the significant trends:
- Cloud Adoption: More businesses are migrating to cloud-based solutions for flexibility and reduced infrastructure costs.
- Integration of AI and Machine Learning: Enhanced capabilities in data processing and predictive analytics offer new insights.
- Focus on Real-Time Data Processing: Organizations require instant data insights to make timely decisions.
- Increased Emphasis on Security: Protecting sensitive data is crucial as regulations around data privacy tighten.
Data-Driven Analysis
Metrics and Criteria for Evaluation
To effectively evaluate data warehouse solutions, enterprises should consider multiple metrics and criteria:
- Performance: Assess speed and efficiency in query processing.
- Scalability: Ensure capability to scale with growing data demands.
- Cost-effectiveness: Estimate total cost of ownership, including licensing, hardware, and operational costs.
- Ease of Use: Evaluate user interface and accessibility of data for non-technical users.
- Integration Capabilities: Analyze how easily the solution can integrate with existing systems and tools.
Comparative Data on Leading Software Solutions
Several data warehouse solutions are prevalent today. Here, we compare a few:
- Amazon Redshift: Known for its high performance and scalability, it supports large amounts of data and integrates well with AWS services.
- Google BigQuery: Offers serverless architecture and real-time analytics, ideal for businesses looking for effortless data management.
- Snowflake: Provides a unique architecture that separates compute and storage, focusing on elasticity and performance.
"The best data warehouse solution aligns with the specific operational needs and growth potential of an enterprise."
Selecting the right data warehouse solution can empower businesses to unlock their data's potential, leading to informed decisions and sustained growth in an increasingly complex environment.
Foreword to Data Warehousing
Data warehousing plays a vital role in the modern enterprise landscape. It serves as a centralized repository for data generated from various sources. As businesses strive to make data-driven decisions, understanding the nuances of data warehousing becomes crucial. The process involves collecting, consolidating, and organizing data, enabling organizations to analyze it effectively for strategic insights.
Definition and Purpose of Data Warehousing
Data warehousing can be defined as the act of collecting and managing data from various sources to provide meaningful business insights. The purpose of implementing a data warehouse is multifaceted. First, it allows the organization to store historical data in an organized manner. This storage is essential for longitudinal analyses, trend identification, and forecasting.
Moreover, data warehousing supports decision-making processes. By presenting data in a user-friendly format, it enables stakeholders to access relevant information quickly. A robust data warehouse architecture can facilitate faster querying and reporting. Ultimately, it helps businesses respond promptly to market dynamics and customer needs.
Historical Context of Data Warehousing
The concept of data warehousing began to take shape in the late 1980s. With the advent of relational databases, companies quickly recognized the need for integrated data storage solutions. Early systems were limited, often focusing on merely collecting transaction data. However, technological advancements led to more sophisticated models. Over time, data warehouses evolved to include various analytical capabilities.
In the 1990s, research by inventors like Ralph Kimball and Bill Inmon influenced the development of data warehousing methods. Kimball proposed a bottom-up approach, focusing on building data marts centered around specific business needs. In contrast, Inmon advocated for a top-down approach, prioritizing a centralized architecture. Both perspectives shaped the way modern enterprises view data storage and usage today. Organizations that embraced data warehousing facilitated enhanced data management and business intelligence, leading to improved competitiveness and efficiency.
Overall, the journey of data warehousing reflects its critical importance in managing data within enterprises, underscoring its relevance in today's data-centric world.
Key Characteristics of Effective Data Warehousing Solutions
The landscape of data warehousing is constantly evolving. With an increased emphasis on data-driven decision-making, identifying the key characteristics of effective data warehousing solutions is crucial for modern enterprises. These characteristics determine how well a solution can support business requirements, adapt to changing demands, and safeguard sensitive information. Addressing these key aspects helps organizations streamline their data processing and analytics capabilities, leading to more effective insights and strategic decisions.
Scalability and Flexibility
Scalability refers to a data warehouse system’s ability to accommodate growth in data volume, users, and processing demands. It becomes especially important as businesses produce more data or add to their user base. A scalable warehouse can efficiently manage and store increasing amounts of information without compromising performance.
Flexibility complements scalability, allowing the system to adjust features and resources based on varied business needs. Organizations may experience changes in data complexity or user requirements, and a flexible data warehouse can seamlessly adapt to these shifts. The following elements highlight the importance of scalability and flexibility:
- Handling Growth: As companies expand, the amount of data produced can double or triple. A scalable solution ensures there is no service interruption.
- Customizability: Businesses often have unique requirements. Flexible solutions allow organizations to customize their platform according to specific analysis needs.
- Cost Efficiency: Scalable warehouses provide a cost-effective way to grow. Instead of investing in extensive resources, enterprises can manage expenses according to their actual usage.
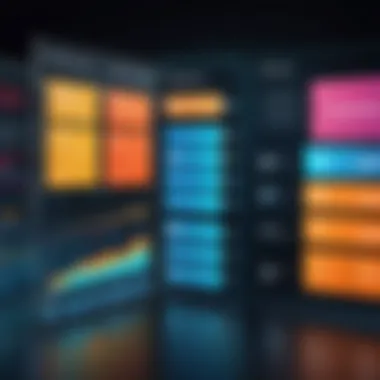
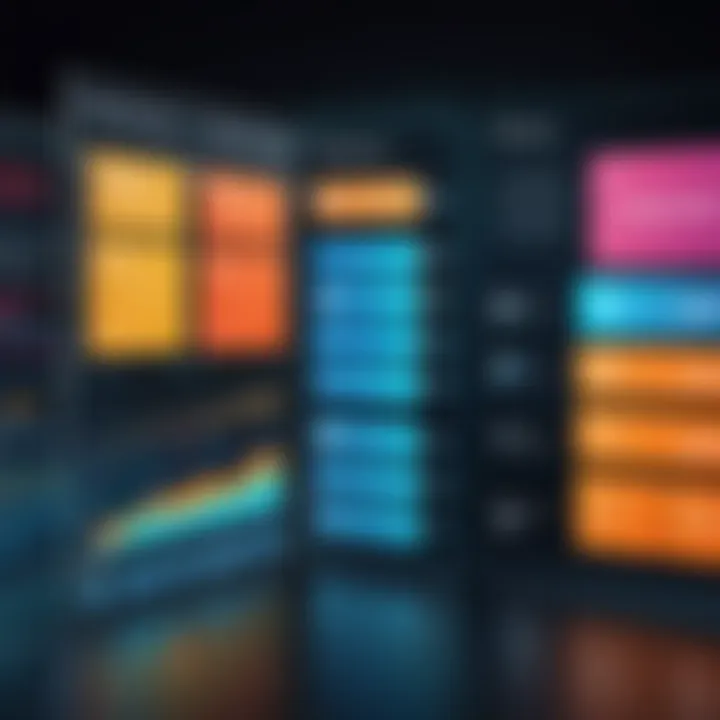
Performance Metrics
In data warehousing, performance metrics are critical to understanding how well the system meets business objectives. Key performance indicators (KPIs) often include query response times, data loading speeds, and overall efficiency of data processing.
Here are some considerations regarding performance metrics:
- Query Performance: Fast and efficient query performance often distinguishes leading data warehouse solutions. Businesses depend on rapid insights from their data.
- Integration Speed: The ability to quickly integrate and process data from various sources also falls under performance metrics. This can significantly impact the timeliness of business intelligence reports.
- Concurrency: High concurrency capacity allows multiple users to operate simultaneously without performance degradation, which is vital in a large enterprise environment.
Security Features
As data becomes an increasingly valuable asset, the importance of security features within data warehouses cannot be overstated. Adequate security measures protect sensitive information from breaches and unauthorized access. Key components of data warehouse security include:
- Data Encryption: Encrypting data both at rest and in transit helps to secure sensitive information.
- Access Controls: Implementing robust access controls can prevent unauthorized users from accessing sensitive data.
- Auditing and Compliance: Compliance with industry regulations is necessary for many organizations. Effective auditing measures ensure that data handling practices meet legal standards.
Evaluating the Best Data Warehouse Solutions
Evaluating data warehouse solutions is a crucial step for any enterprise aiming to utilize data effectively. It allows organizations to align their choice of a data warehouse with their specific needs and business objectives. The selection process involves assessing various factors that contribute to the overall performance, usability, and financial viability of the solutions available in the market. When organizations engage in a thorough evaluation, they can identify solutions that not only offer robust functionality but also integrate seamlessly into their existing workflows.
Key elements to consider when evaluating these solutions include:
- Performance metrics such as query speed and data retrieval times, which are critical for operational efficiency.
- Cost considerations that encompass both initial setup expenses and ongoing operational costs, ensuring that the chosen solution fits within budget limits.
- User experience and support, which consist of the interface's intuitiveness and the availability of help and resources.
- Scalability, to ensure that the solution can grow alongside the organization.
By carefully considering these aspects, businesses can make informed decisions that facilitate better data management, support strategic objectives, and enhance overall business intelligence capabilities.
Cost Considerations
Cost considerations play an essential role in the decision-making process for any enterprise looking to implement a data warehouse solution. Companies must analyze various costs, including initial setup fees, licensing models, hardware requirements, and ongoing operational expenses. It is important to note that not all data warehouse solutions have transparent pricing models, which can lead to unexpected costs later.
- Initial Setup Costs: Many solutions require significant investment upfront for infrastructure. This can include both hardware and software expenditures.
- Licensing Models: Some solutions may charge on a subscription basis, while others might offer pay-per-use options. Comparing these models is vital to understanding the long-term financial implications.
- Operational Costs: Ongoing costs should also include maintenance, support, cloud storage fees, and potential costs for additional user licenses.
- Hidden Costs: Businesses should be aware of potential hidden costs related to performance optimization or training staff to use the new system effectively.
By evaluating these cost factors carefully, decision-makers can choose a data warehouse solution that aligns with their financial constraints while still serving their operational needs.
User Experience and Support
User experience and support are critical factors in the successful adoption of a data warehouse solution. A positive user experience can directly impact productivity and satisfaction among team members. Thus, evaluating the interface and support structure of different options should not be overlooked.
- Interface Usability: Solutions should provide an intuitive interface that simplifies data management tasks. A complex or disorganized user interface may lead to frustration and inefficiencies.
- Support Availability: Access to reliable customer support is crucial. Organizations need to find solutions with robust support options, including training, documentation, and live assistance.
- Community Support: Some platforms have active user communities that contribute tutorials, forums, and publicly available resources. This can be beneficial for troubleshooting and learning best practices.
- Integration Support: User experience also includes the ease with which the data warehouse solution integrates with other tools and systems in use. Robust integration capabilities streamline workflows and enhance functionality.
Overall, focusing on user experience and support during the evaluation process can lead to a smoother implementation and better overall returns on investment.
Top Data Warehouse Platforms Explored
In today's data-driven landscape, businesses need robust solutions to manage vast amounts of information efficiently. This section delves into leading data warehouse platforms, highlighting their significant features, advantages, and essential considerations for enterprises looking to enhance data management strategies. Understanding these platforms helps organizations select the right tool, ensuring optimal performance and value for their specific needs.
Amazon Redshift
Amazon Redshift is a fully managed data warehouse service that offers fast query performance. Its architecture allows it to scale easily, accommodating growing data while maintaining efficiency. The use of columnar storage helps reduce the amount of I/O needed for queries, providing significant speed advantages over traditional row-based systems.
Key features of Amazon Redshift include:
- Scalability: It can handle petabyte-scale data, making it suitable for large operations.
- Cost Efficiency: Pay-as-you-go pricing lowers the total cost of ownership, especially for businesses with fluctuating workloads.
- Integration: Seamless connections with other Amazon Web Services enhance its capabilities, allowing for advanced analytics.
Considerations: Redshift's performance can depend on the right cluster configuration. Understanding query patterns and workload management are crucial for taking full advantage of its features.
Google BigQuery
Google BigQuery is a serverless data warehouse solution designed for big data. It excels in real-time analytics and offers features that streamline the data processing workflow. With its ability to run ANSI SQL queries across massive datasets, it is particularly favored by organizations needing quick insights.
Main attributes include:
- Flexibility: Allows users to focus on analysis without managing infrastructure.
- Integrated Machine Learning: Built-in ML capabilities provide advanced analytical options directly within the platform.
- Storage and Compute Separation: Users can scale storage and compute resources independently, optimizing costs based on usage.
Considerations: Although easier to use, there may be a learning curve related to its infrastructure compared to traditional data warehouses.
Microsoft Azure Synapse Analytics
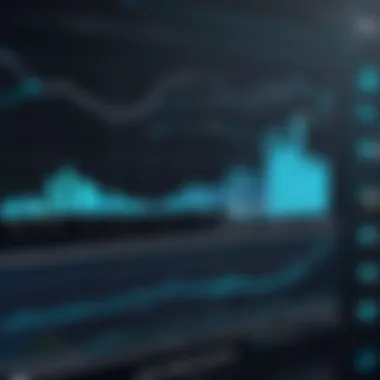
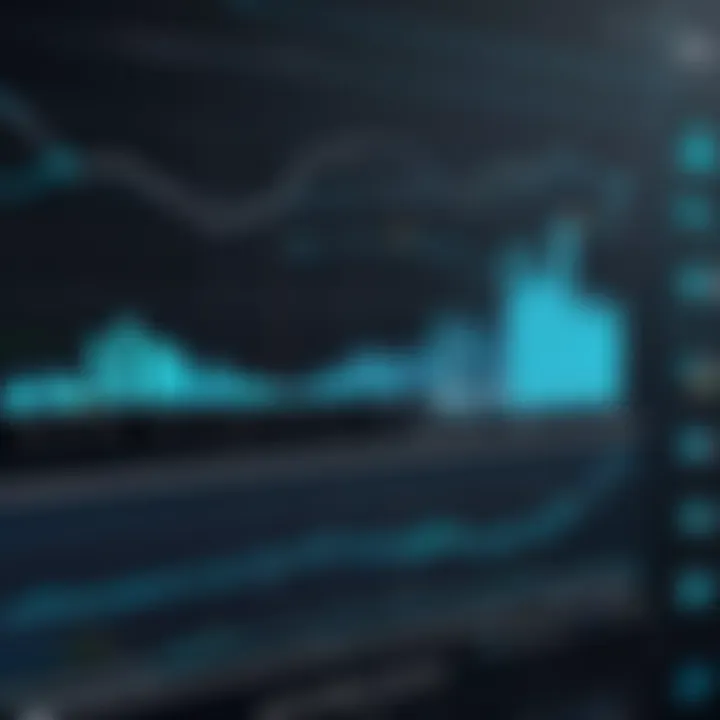
Microsoft Azure Synapse Analytics, formerly known as Azure SQL Data Warehouse, integrates big data and data warehousing. It offers powerful analytics services and the ability to analyze data in real-time. The platform promotes collaboration through integration with tools like Microsoft Power BI and Azure Machine Learning.
Notable features consist of:
- Unified experience: Combines data integration, warehousing, and big data analytics into one service.
- Security: Robust data protection with advanced threat detection and management features.
- Hybrid capabilities: Supports on-premises data sources, offering flexibility in diverse environments.
Considerations: Organizations should assess their existing Microsoft infrastructure to fully leverage the benefits of Azure Synapse Analytics.
Snowflake
Snowflake is a cloud-based data warehouse designed with flexibility and scalability at its core. Its architecture separates storage and compute, enabling users to adjust resources to meet varying workloads without impacting performance.
Key points include:
- Concurrency: Supports thousands of concurrent users and queries without performance degradation.
- Data Sharing: Offers secure and seamless data sharing capabilities across organizations.
- Multi-Cloud Support: Operates on major cloud services, providing flexibility in infrastructure choice.
Considerations: Optimal performance requires a good understanding of costs related to storage versus compute usage, as they are billed separately.
Oracle Autonomous Data Warehouse
Oracle Autonomous Data Warehouse utilizes machine learning to automate database management. This platform focuses on providing high performance and reliability while simplifying administrative tasks. It is particularly beneficial for enterprises looking for low maintenance combined with power.
Central aspects include:
- Self-Management: Automates updates, backups, and scaling, allowing IT teams to focus on more strategic tasks.
- High Performance: Optimized for complex queries and large datasets.
- Security: Comprehensive data protection measures ensure strong governance and compliance.
Considerations: Enterprises should evaluate if they require the extensive features this platform offers, as it might be more complex than simpler solutions.
Overall, each platform offers distinct advantages tailored for various business needs. Assessing organizational goals, data growth, and specific use cases will facilitate the choice of the most suitable data warehouse solution.
Comparative Analysis of Data Warehouse Solutions
In a landscape where data drives decision-making, the comparative analysis of data warehouse solutions becomes crucial for organizations aiming to maximize their operational efficiency. Understanding the nuances among different solutions helps businesses identify what aligns best with their needs. This section evaluates three major components: performance, pricing structures, and feature sets. By delving into these areas, decision-makers can weigh options and choose a data warehouse that not only fits their budget but also addresses their strategic goals.
Performance Comparison
Performance is often the foremost consideration when selecting a data warehouse solution. It refers to its capability to handle large volumes of data, process queries rapidly, and support simultaneous user access. Slow performance can hinder operational efficiency and affect business intelligence efforts. Factors influencing performance include:
- Query speed: How fast can the system process and return results?
- Concurrent users: Can the solution support multiple users without degradation in performance?
- Data ingestion: How quickly can data be loaded into the warehouse for analytics?
Leading data warehouse platforms like Amazon Redshift and Google BigQuery leverage distributed computing to enhance performance, but each has unique strengths and weaknesses. For example, Google BigQuery, with its serverless architecture, excels in handling massive datasets and complex queries on-the-fly, while Redshift might require more careful tuning for optimal performance.
Pricing Structures
Pricing structures vary widely across data warehouse solutions, often influenced by factors such as data storage needs, computational requirements, and user access levels. Understanding these structures is critical in evaluating total cost of ownership.
Key considerations when analyzing pricing structures include:
- Pay-as-you-go vs. fixed pricing: Some solutions operate on a pay-as-you-go model, allowing businesses to pay only for resources used, which can be beneficial for variable workloads. Others, like Oracle Autonomous Data Warehouse, offer more predictable fixed pricing.
- Additional costs: Examine potential hidden costs associated with data transfer, storage overhead, or support fees.
- Discount models: Many providers offer discounts at scale, which can lower costs significantly for larger organizations.
A thorough understanding of these pricing structures can lead to significant cost savings and help organizations adapt their data warehousing strategy as their needs evolve.
Feature Set Comparison
The feature set of a data warehouse solution directly impacts its usability and operational fit. Features encompass a broad range of functions, from data modeling capabilities to compliance adherence and integration with existing tools. Evaluating features includes:
- Integration capabilities: Compatibility with various data sources, such as SQL databases, cloud services, and third-party applications.
- Data transformation tools: Built-in ETL (Extract, Transform, Load) functionalities vs. reliance on external tools like Talend or Apache NiFi.
- Analytics and reporting tools: Ease of use in creating dashboards and generating reports for end-users.
For example, Snowflake stands out with its robust integration capabilities and support for a multi-cloud environment, making it suitable for organizations with diverse infrastructures. Meanwhile, Microsoft Azure Synapse Analytics focuses on its deep integration with other Microsoft tools, catering effectively to businesses embedded in the Microsoft ecosystem.
"The depth of analysis of performance, pricing, and features ensures that organizations can make informed decisions rather than relying on superficial comparisons."
A comparative analysis equips businesses with a profound understanding of their options, leads to more strategic investment in data warehousing solutions, and ultimately enhances the organization’s ability to turn data into actionable insights.
Case Studies on Implementing Data Warehouse Solutions
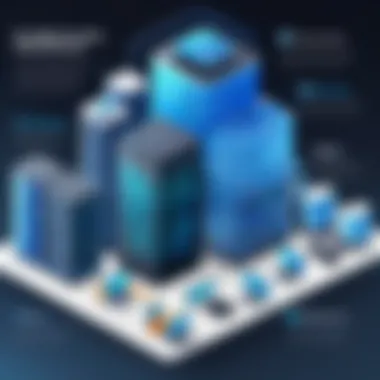
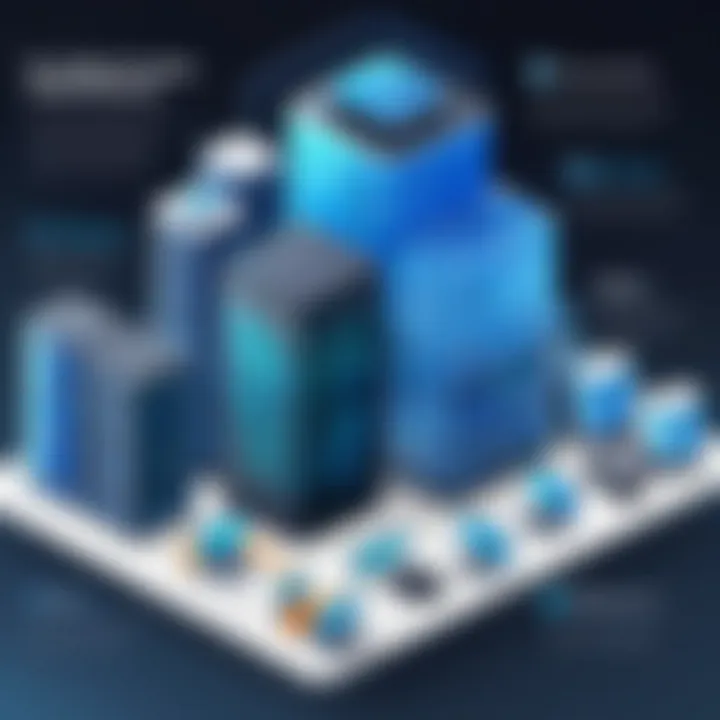
Understanding real-world applications of data warehouse solutions is essential for businesses. Case studies serve as critical references, showcasing success stories and the challenges faced during implementation. They allow decision-makers to glean valuable lessons from the experiences of others, facilitating informed choices for their own enterprises. By examining these detailed examples, organizations can appreciate the nuances of deployment, the specific benefits realized, and any pitfalls to be wary of.
The importance of case studies extends beyond mere illustration; they provide concrete evidence for the efficacy of a solution. In this section, we will explore two influential case studies that highlight the effectiveness of Amazon Redshift in business intelligence and Google BigQuery in real-time analytics. Each example will reveal unique insights while emphasizing the alignment of data warehousing solutions with specific organizational needs.
Use Case of Amazon Redshift in Business Intelligence
Amazon Redshift has emerged as a prominent choice for companies seeking robust business intelligence capabilities. One notable use case can be found in a large retail organization that wanted to improve its sales analytics. The company faced challenges with data silos, which hampered its ability to conduct thorough analyses and make data-driven decisions.
Leveraging Amazon Redshift, the organization integrated disparate data sources into a unified data warehouse. The platform's scalability allowed it to handle vast volumes of sales data while providing rapid query performance. As a result, the retail business was able to generate analytics reports in real-time, enhancing inventory management and sales forecasting processes.
The benefits realized by this organization extended beyond improved analytics. By employing Amazon Redshift, the company saw a marked increase in collaboration among teams, as data became more accessible. Furthermore, the overall cost of ownership was reduced. They shifted from a traditional, on-premises solution to a cloud-based model, enabling them to pay only for what they used. This transformation illustrates how Amazon Redshift can be a strategic asset for companies focused on enhancing their business intelligence capabilities.
Google BigQuery Applications in Real-Time Analytics
Google BigQuery stands out in use cases revolving around real-time analytics. A prominent media company utilized BigQuery to optimize its advertising campaigns and audience analysis. The organization needed to process large amounts of clickstream data in near real-time to derive actionable insights quickly.
Through Google BigQuery, the media business achieved a seamless integration of its data pipelines, allowing it to run complex queries across massive datasets without concerns surrounding performance. The ability to scale on-demand meant that the company could adjust its resources dynamically, depending on traffic and campaign needs.
Moreover, the near-instant querying capability empowered their analytics team to act swiftly on data insights. This responsiveness to real-time data led to better-targeted ads, ultimately improving both conversion rates and return on investment. Such a case study underscores not just the technical prowess of Google BigQuery, but also how it catalyzed significant business advantages by empowering timely decision-making.
Through these examinations, businesses can derive direction and inspiration for their own data warehousing journeys.
Future Trends in Data Warehousing
As the landscape of data management evolves, understanding the future trends in data warehousing becomes crucial for organizations aiming to remain competitive. This section will focus on the importance of recognizing these trends, the benefits they offer, and the considerations businesses must keep in mind when adapting to these changes. Among the most significant trends are the increasing reliance on cloud computing and the growing integration of artificial intelligence.
The Role of Cloud Computing
Cloud computing has fundamentally reshaped the approach to data warehousing. It provides organizations with the ability to store and process vast amounts of data more efficiently and at a lower cost. The scalability of cloud solutions allows businesses to effortlessly adjust their storage needs in response to growing data volumes without significant infrastructure investments.
Moreover, cloud storage enhances collaboration. It allows teams to access data from anywhere, which is especially beneficial in today’s remote work environments. Security measures like encryption and regular updates from cloud service providers further bolster user confidence, making this a preferred option for many.
*In a modern enterprise, the cloud serves not only as a repository but also as a dynamic workspace where data flows seamlessly.
Key benefits of cloud computing in data warehousing include:
- Cost Efficiency: Pay-as-you-go models minimize upfront costs and reduce the need for large-scale hardware purchases.
- Automatic Scalability: Resources can be quickly scaled up or down based on demand, optimizing operational costs.
- Enhanced Security: Regular updates and strong encryption capabilities help protect data against breaches.
Integration with Artificial Intelligence
Artificial intelligence (AI) is becoming increasingly vital in data warehousing. Its integration facilitates advanced analytics, which can provide insights far beyond basic reporting. AI algorithms can identify patterns in large datasets, enhance data quality, and even automate routine tasks, allowing employees to focus on more strategic work.
Furthermore, AI-powered tools can improve decision-making processes by delivering predictive analytics and real-time insights. Such effectiveness not only enhances operational efficiency but also drives innovation within organizations that adopt these technologies.
Businesses must consider the following while integrating AI into their data warehousing solutions:
- Skillset Requirements: As AI tools become more prevalent, there's a growing need for IT professionals who understand both data warehousing and AI technologies.
- Data Governance: The integration of AI raises questions about data handling and compliance. Organizations must ensure they maintain robust data governance frameworks.
- Customization Potential: Many AI solutions offer tailored approaches to meet specific needs, which may require thorough testing to determine fit.
The trends of cloud computing and artificial intelligence are reshaping the data warehousing industry. As they continue to evolve, staying informed will be key for businesses aiming to harness their full potential.
Epilogue and Recommendations
The conclusion and recommendations section serves as a vital component of the discourse surrounding data warehouse solutions. This article has outlined the intricate elements that influence the efficacy of such systems for modern enterprises. The essence of choosing the right data warehouse solution revolves around various factors, including performance, scalability, cost-effectiveness, and alignment with business objectives.
By synthesizing the information presented throughout this article, decision makers can appreciate the nuances involved in selecting the optimal data warehouse. Each enterprise has specific needs that dictate which features are essential. It is important to understand that no one-size-fits-all solution exists. Hence, weighing the benefits against the specific context of the business is crucial for making informed decisions.
"The right data warehouse solution maximizes operational efficiency, ensuring that data is not just stored but also managed for actionable insights."
Final Thoughts on Choosing a Data Warehouse Solution
Choosing a data warehouse solution necessitates a multi-faceted approach. Initially, an organization must evaluate its data strategy, considering the types of data processed, the volume of transactions, and integration with existing systems. A clear grasp of these requirements ensures that the selected solution can support current and future needs.
Additionally, it is beneficial to engage with stakeholders within the organization. Understanding their objectives, pain points, and expectations can help delineate the functionalities that the data warehouse must deliver. Ultimately, testing the solution through trials or demos can offer valuable insight into how well it meets the enterprise’s requirements.
Recommendations Based on Business Size
Recommendations for choosing a data warehouse solution often hinge on the size of the business. Smaller enterprises might prioritize cost-effectiveness and ease of use, leaning towards platforms like Amazon Redshift or Google BigQuery, which provide flexible pricing structures. These solutions allow for scalability without the burden of upfront infrastructure costs.
Medium to large businesses may require more robust features. They generally benefit from comprehensive platforms such as Microsoft Azure Synapse Analytics or Snowflake. These solutions offer deeper analytics capabilities, enhanced support for complex queries, and greater integration potential with existing IT landscapes.
In summary, the recommendation is to align the data warehouse choice not just with current needs but also with anticipated growth and evolving technological landscapes. By doing so, businesses can pave the way toward a more data-driven future.