Finance Master Data Management: Key Insights for Leaders
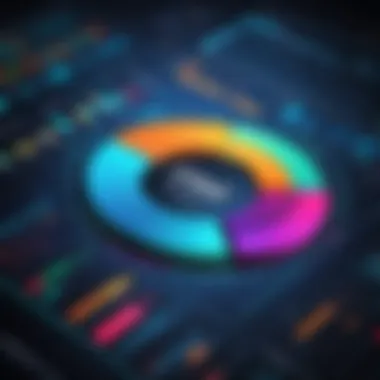
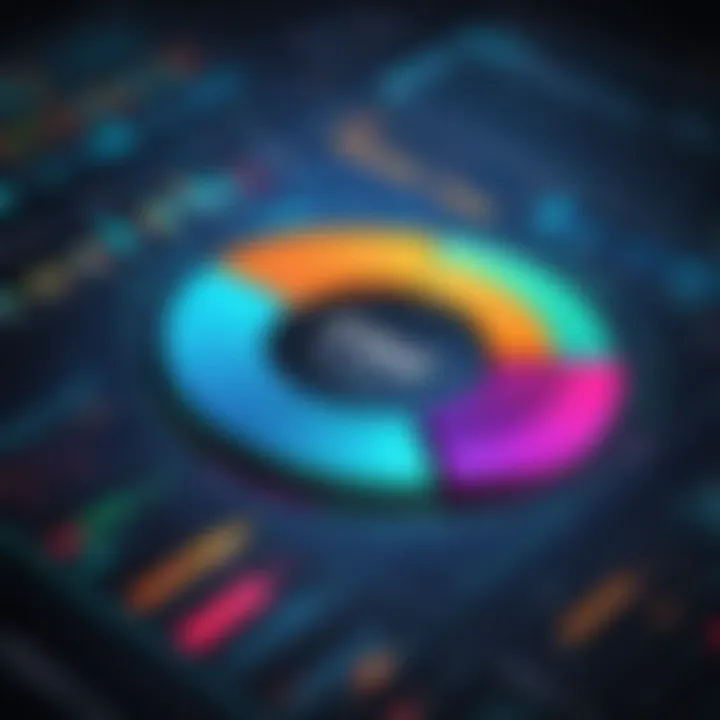
Intro
In today’s hyper-connected world, the accuracy of financial data holds the key to unlocking a company's potential. Finance master data management (FMDM) stands as a cornerstone in ensuring this accuracy, providing a structured approach to handling financial data across an organization. From optimizing operational efficiency to enhancing decision-making, the relevance of FMDM cannot be overstated. This article will take you through the intricate facets of finance master data management, exploring its importance, challenges, and best practices that shape its governance.
Software Category Overview
Purpose and Importance
Finance master data management software is designed to centralize the management of critical financial data. This software facilitates consistency and accuracy across all departments within an organization. In essence, it acts as a single source of truth. Imagine the chaos of having multiple, disjointed financial records—this not only complicates audits but also hampers strategic planning. A robust finance master data management system allows companies to leverage their financial data effectively, leading to improved compliance, enhanced performance, and smarter, data-driven decision-making.
Current Trends in the Software Category
In recent years, there has been a noticeable shift towards cloud-based solutions in financial data management. Businesses are increasingly adopting software that offers scalability, accessibility, and real-time analytics. Emerging technologies, such as artificial intelligence and machine learning, are being integrated into finance master data management tools, helping organizations to make sense of large volumes of data more efficiently. Here are a few current trends:
- Automation: Reducing manual intervention to minimize errors.
- Data Integration: Seamless synchronization with other business systems, like ERP and CRM.
- Advanced Analytics: Utilizing data insights for predictive modeling and what-if scenarios.
Data-Driven Analysis
Metrics and Criteria for Evaluation
Evaluating finance master data management systems isn’t just about the features; it’s about how these features align with the needs of your entity. Metrics for evaluation could include:
- Data Quality Indicators: Accuracy, consistency, and completeness of data.
- User Adoption Rates: Percentage of employees actively using the system.
- Process Improvement: Reduction in time and resources for data management tasks.
- Regulatory Compliance: Adherence to financial regulations and standards.
These metrics can help stakeholders determine whether the chosen software meets the expectations for operational efficiency and data governance.
Comparative Data on Leading Software Solutions
With several players in the finance master data management arena, it’s vital to comprehend how each solution stacks up. While there are various systems available, a few stand out based on features, user feedback, and performance metrics. Consider solutions like SAP Master Data Governance or Informatica Master Data Management. Each comes with its set of strengths and weaknesses, often hinging on aspects such as integration capabilities, support, and pricing structures.
"The cornerstone of a successful business lies not in just having data, but utilizing it efficiently to foster growth and innovation."
The choice of the right software can propel organizations to new heights, making the analysis of product offerings crucial in any decision-making process.
Prolusion to Finance Master Data
In today's fast-paced financial landscape, the role of finance master data cannot be overstated. As organizations strive for efficiency and accuracy, the data that underpins financial operations becomes critical. This section sheds light on the importance of finance master data, describing its definitions, significance, and impact on decision-making within enterprises.
Defining Finance Master Data
Finance Master Data refers to the essential data sets that organizations base their financial transactions, reporting, and analysis upon. This encompasses a variety of critical elements such as account information, cost centers, and organizational structures. Finance master data acts as a backbone supporting all financial activities—from budgeting to forecasting and financial reporting.
Consider a financial analyst using data to generate a report. If the foundation—the finance master data—is inconsistent or inaccurate, the analysis becomes flawed. That can lead to misinformed decisions, allocating resources inefficiently, or overlooking potential revenue streams. Therefore, defining finance master data accurately is the first step towards ensuring robust financial operations.
Importance of Master Data in Finance
The importance of finance master data extends beyond its immediate functions. Consolidating and managing this data effectively contributes to various benefits, including:
- Consistency Across Departments: When finance master data is uniform, it reduces the likelihood of misunderstandings. For instance, when different departments refer to the same cost center in reports, it ensures that everyone is on the same page.
- Enhanced Decision-Making: Access to accurate finance master data enables stakeholders to craft informed decisions. Data-backed insights lead to strategic planning that can result in optimized investments and improved bottom lines.
- Regulatory Compliance: Organizations need to adhere to financial regulations, such as GAAP or IFRS. Having streamlined finance master data helps in preparing reports that meet compliance requirements more efficiently.
- Operational Efficiency: Regular reliance on accurate master data minimizes time spent on resolving discrepancies, allowing financial teams to focus on strategic initiatives rather than data correction tasks.
In summary, finance master data is fundamental in driving precision and accountability in financial management. Organizations that prioritize the definition and management of such data position themselves for a competitive edge in the market.
Components of Finance Master Data
In the intricate landscape of finance master data management, understanding the components is akin to assembling the pieces of a complex puzzle. Each element plays a crucial role in ensuring that data is accurate, accessible, and relevant to decision-making processes. Without a detailed grasp of these components, organizations risk running into a maze of data inconsistencies that can lead to poor strategic outcomes. Therefore, this section focuses on core financial entities and dimension data, both vital to mastering the world of finance data.
Core Financial Entities
Accounts
When delving into finance master data, accounts emerge as a foundational pillar. They represent categories through which financial transactions are recorded, forming the backbone of financial reporting for any organization. Each account is classified to ensure an organized method of tracking revenues, expenses, assets, and liabilities.
A notable characteristic of accounts is their ability to segment data into understandable chunks, making it easier for professionals to analyze financial health. This systematic approach is why accounts remain a widely favored option in finance master data conversations.
However, it’s wise to note that the unique feature of accounts—being open and flexible to adjustments—can pose challenges. If not properly governed, these adjustments may lead to notable discrepancies, muddling the financial picture. The advantages lie in intuitive tracking, whereas the disadvantages could stem from over-reliance on manual changes, opening the door for errors.
Departments
The structure of any organization is typically mirrored in its departments. Departments are essential for defining how various units contribute to the overall financial landscape and help clarify financial accountability and responsibility. Each department plays a different role, with their data showcasing how spending and resources align with strategic objectives.
Departments also provide a critical layer of granularity. This characteristic is particularly useful, as it not only assists in the allocation of budgets but also enhances performance evaluations. In many instances, businesses rely heavily on departmental data to guide financial decisions, making it a robust choice in the context of finance master data.
Yet, the unique feature of departmental breakdown can backfire if silos emerge. If departments don’t share data openly, decision-making can be stymied, creating gaps in understanding. The benefits of a comprehensive departmental approach are significant, but keeping the channels of communication open is vital to avoid pitfalls associated with fragmented information.
Sub-ledgers
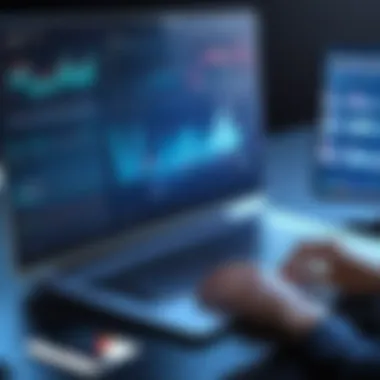
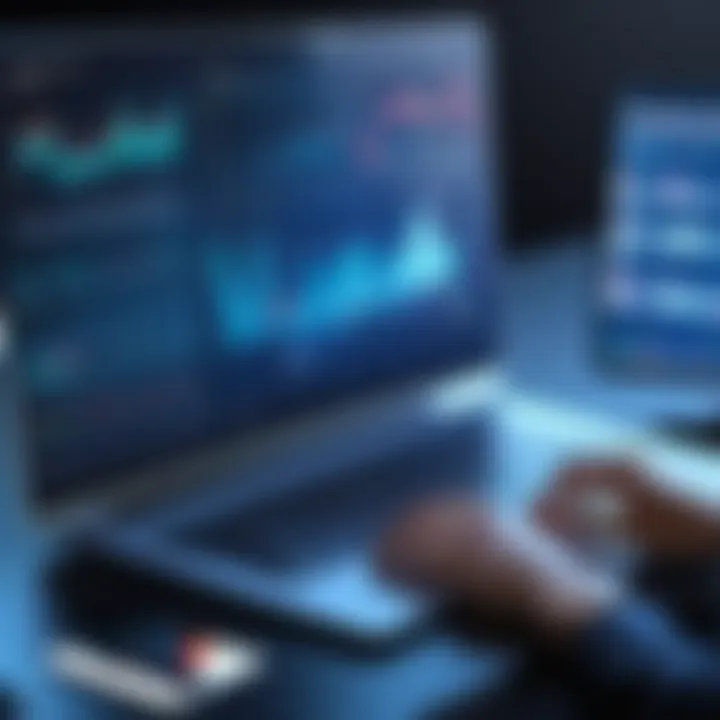
A deeper dive into finance would be incomplete without addressing sub-ledgers. These are specialized records that provide insights into individual transactions without losing sight of the bigger financial picture. Sub-ledgers offer detailed tracking of various activities, such as accounts payable and receivable or inventory, thus acting as supportive documentation for main ledgers.
One key characteristic of sub-ledgers is their ability to ensure that detailed data is accessible while keeping the main financial statement concise. This practice is invaluable for audits, as the trail of transactions can be easily followed. The unique feature lies in their detailed nature; however, if not adequately maintained, sub-ledgers can become unwieldy, leading to confusion rather than clarity.
Dimension Data
Dimension data adds another layer of depth to understanding finance master data. It encapsulates the contextual elements that help organizations analyze trends, performance, and opportunities.
Time Periods
Time periods are critical in the world of finance, acting as methods of measuring performance over distinct intervals. They help in reporting practices by ensuring that financial data can be categorized effectively, facilitating year-over-year comparisons, quarterly assessments, and monthly forecasts. This segmentation aids organizations to pinpoint seasonal trends in financial performance.
As a key characteristic, time periods lend structure to financial evaluations, effectively framing the data in a way that's both understandable and actionable. A unique feature is their availability in various formats—monthly, quarterly, or annually—allowing organizations to select the granularity that suits their needs. The downside, though, is that overemphasis on specific time frames can sometimes overshadow longer-term strategic trends.
Geographical Segments
With financial data, geography plays a significant role. Geographical segments offer insight on how various locations contribute to an organization's overall financial standing. This stratification can reveal opportunities or challenges specific to markets and enables firms to tailor strategies accordingly.
A standout characteristic of geographical segmentation is its ability to expose the diverse operational impacts across different regions. This feature paves the way for targeted decision-making, making it a favored choice in financial analyses. However, the increased complexity can sometimes overwhelm analysts, creating challenges in ensuring data integrity across multiple regions.
Product Categories
Lastly, product categories delineate the various goods or services that a business offers, showcasing their performance relative to one another. This classification allows organizations to track which products are generating revenue and which ones may be faltering.
The primary characteristic of product categories is their role in performance measurement; it aids in responding to market demands promptly. Their unique feature includes the ability to align product offerings with customer preferences and market trends, driving strategic actions. Yet, on the flip side, over-categorization can lead to analysis paralysis, where the richness of data becomes a hindrance rather than a helping hand.
Understanding these components—in all their complexity and utility—equips organizations to harness their finance master data most effectively. Integral to financial reporting and decision-making, these elements not only enhance organizational efficiencies but form the crux of strategic financial management.
Challenges in Managing Finance Master Data
Managing finance master data is more than just tidying up spreadsheets. It’s about ensuring that the numbers telling a company’s story are both accurate and reliable. When the foundation of data is shaky, everything built upon it can tilt. Here, we’ll unpack some pressing challenges that organizations encounter in this crucial area. The effective control of finance master data not only enhances operational efficiency but also fortifies the decision-making process. Understanding these hurdles is a step toward mastering data management.
Data Quality Issues
Inconsistencies
Inconsistencies can feel like trying to piece together a jigsaw puzzle with mismatched pieces. One department may report revenue figures that vary drastically from another. This can stem from differences in how data is captured or processed, leading to conflicting information. The key characteristic here is the variation in data representation across systems, making it tricky to obtain a single version of the truth. Tackling inconsistencies is critical for any organization aiming for cohesive financial reporting. If done correctly, it propels the accuracy of financial insights and ultimately leads to better strategic decisions.
Disadvantageously, it often requires significant time and manpower to identify and rectify these inconsistencies. Furthermore, relying on manual checks can introduce human errors which often muck everything up.
Duplications
In the realm of finance, duplicates are like weeds in a garden; they sprout up when least expected, consuming valuable resources. Duplicate entries often arise due to different systems or departments entering the same data independently. The key characteristic of duplications is the redundancy they create, which skews reports and analyses. It can sap an organization's focus and effectiveness, snowballing into larger operational inefficiencies.
For this article, recognizing the unique feature of duplications comes into play: they dilute the quality of data and inflate storage costs. An organization that can eliminate duplicated data not only clears up its datasets but also simplifies its financial analysis, leading to cleaner insights. But removing duplicates isn’t always straightforward; it requires a systematic approach to identify and reconcile them.
Incomplete Records
Incomplete records can haunt financial departments like a ghost that just won’t leave. When records lack crucial information, the financial picture becomes unclear, jeopardizing trust in data. A key characteristic is the absence of essential data points, potentially due to errors during entry or oversight. This absence can lead to critical decisions being based on half-truths.
In this article context, incomplete records illustrate the need for robust data capture processes. The unique feature of such records is that they create gaps in financial reporting making complex analysis nearly impossible. Without a full complement of data, organizations risk making decisions based on partial evidence, which can lead to severe implications down the line.
Siloed Data Sources
Legacy Systems
Legacy systems are often like a pair of well-worn shoes. They might have served the organization faithfully for years, but they’re now showing their age. These outdated systems can severely hinder the flow of finance master data, creating barriers to integration with newer technologies. The key characteristic of these systems is their incompatibility with modern applications, leading to inefficiencies and increased chances of error.
In our discussion about this article, highlighting legacy systems serves to underscore the need for modernization in data management. They lack the flexibility required for today’s fast-paced business environment. Their unique feature lies in the data silos they create, isolating valuable financial data across various departments. This can effectively grind processes to a halt, making it cumbersome for professionals to get their hands on accurate and timely numbers.
Disparate Applications
Disparate applications might seem like a convenient fix for separate data management needs, but as time rolls on, they can morph into a tangled web of confusion. When different departments use different software, data can become fragmented, hindering comprehensive financial analysis. The key characteristic here is the lack of synchronization across these applications. Missing data transfer protocols often lead to discrepancies.
In terms of this article, focusing on disparate applications is critical because they can stifle efficient communication. The unique feature of such setups can complicate financial reporting - when data isn’t universally accessible, it becomes arduous to derive holistic insights. In many cases, companies may find themselves deciding whether to invest in integration solutions or face the consequences of inefficiency and increased costs.
Best Practices in Finance Master Data Management
In the realm of finance master data management, best practices serve as the bedrock upon which successful systems are built. These practices not only facilitate the efficient handling of financial information but also ensure the integrity and reliability of such data. Adopting best practices is like having a roadmap that guides organizations through the intricacies of finance master data, helping them navigate potential pitfalls.
A comprehensive approach to managing master data encompasses various elements that highlight its intrinsic value. By implementing these approaches, organizations can expect enhanced operational efficiency, informed decision-making, and compliance with regulatory standards. Below, we delve into key best practices that can propel finance master data management efforts.
Establishing Governance Frameworks
The first step in managing finance master data effectively is establishing robust governance frameworks. These frameworks define how data is managed across the organization, outlining policies, processes, and standards that need to be adhered to. Governance is not just a set of rules; it embodies a culture of responsibility and accountability towards financial data management.
Roles and Responsibilities
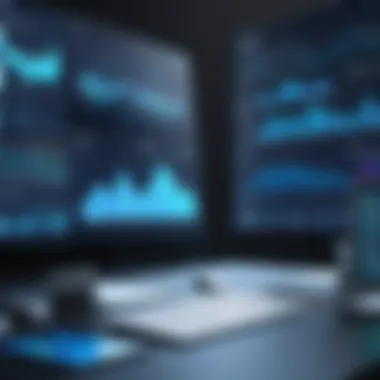
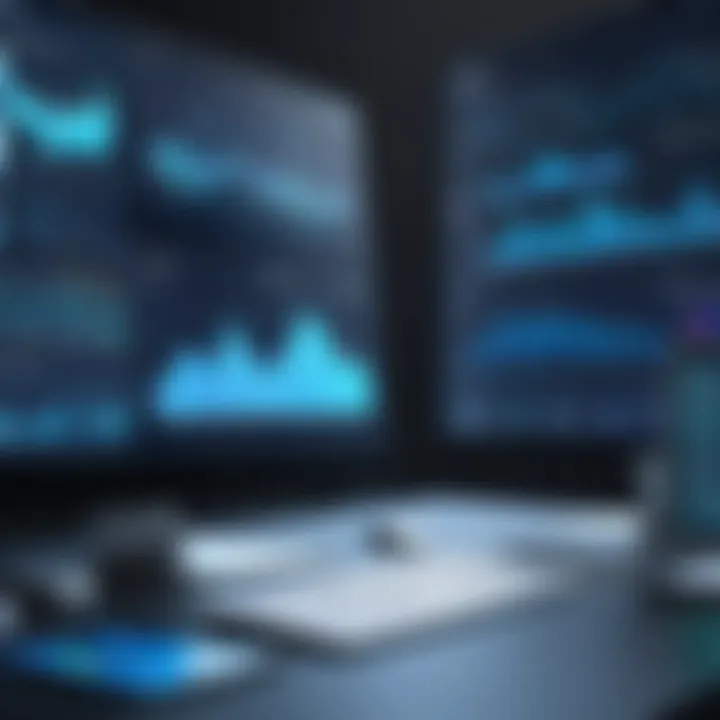
The clear delineation of roles and responsibilities is a cornerstone of any governance framework. Identifying who is responsible for what in the realm of finance master data helps reduce ambiguity and creates a structured environment conducive for data management. This clarity minimizes the risk of oversight, ensuring that every piece of data has a designated owner.
Key Characteristics:
- Accountability: Assigning specific duties fosters a sense of ownership and vigilance among staff.
- Clarity: Reduces confusion about data management responsibilities.
Thus, having well-defined roles is a no-brainer for organizations aspiring for data excellence. The unique feature lies in aligning these responsibilities with organizational goals, thereby creating not just a compliance checklist but a proactive approach to data stewardship.
"When roles are clearly outlined, the path to data integrity becomes less cumbersome."
Data Stewardship
Data stewardship focuses on the careful management of data assets, ensuring they are accurate, accessible, and trustworthy. It acts as a guiding principle in the governance framework, promoting best practices and criteria for maintaining data quality.
Key Characteristics:
- Accuracy: Data stewards are responsible for ensuring that the financial data is accurate and current.
- Training: They often provide training and resources to team members on data best practices.
The unique attribute of data stewardship is its proactive nature. Stewards don't just react to data issues; they anticipate potential hurdles and mitigate them before they emerge, thus fostering a resilient data management culture. However, this responsibility can often be overwhelming if not properly supported by organizational structures, making it vital to have clear protocols in place.
Implementing Data Quality Protocols
Data quality is paramount in finance master data management. Implementing stringent data quality protocols is essential for maintaining the integrity of financial information. These protocols can drastically reduce errors, ensuring data accuracy and reliability.
Regular Audits
Regular audits are fundamental in verifying that the established data quality protocols are functioning effectively. Conducting audits frequently helps in identifying any discrepancies or inconsistencies before they spiral into larger issues. This proactive approach is integral in maintaining a robust master data management framework.
Key Characteristics:
- Continuous Monitoring: Audits provide an ongoing assessment of data quality, catching problems in real-time.
- Risk Management: They allow for the identification of potential risks associated with data handling.
Regular audits are indeed a wise investment. Their unique advantage lies in fostering a mindset focused on improvement and vigilance. However, they can also be resource-intensive, requiring a dedicated team and time commitment, which some organizations may find challenging.
Validation Processes
The validation process involves verifying the accuracy and integrity of data entries before they are finalized. Implementing validation checks at various stages of data handling contributes significantly to the overall quality of finance master data.
Key Characteristics:
- Real-time Verification: This ensures discrepancies are addressed promptly.
- User Responsibility: Encourages data-entry personnel to be more meticulous in their work.
The unique aspect of validation processes is their adaptability to different data handling situations. They can be tailored to meet specific requirements, thus enhancing their effectiveness in various contexts. However, failure to keep validation criteria updated can thwart their effectiveness, highlighting the need for ongoing review and adaptation.
By navigating the complexities laid out here, organizations can significantly enhance their finance master data management practices, ensuring that they are always several steps ahead in data quality and governance.
Technological Solutions for Finance Master Data
In the landscape of finance, where precision and timeliness reign supreme, relying on robust technological solutions for managing master data is crucial. The right tools not only streamline operations but also enhance the quality and accessibility of financial data. These technological solutions serve as frameworks upon which organizations can build their data strategies, ensuring that information is not just stored but also leveraged effectively for decision-making.
Master Data Management Software
Features to Consider
When looking at Master Data Management (MDM) software, one must keep an eye on the underlying features that define its effectiveness. One key aspect to consider is usability. An intuitive interface ensures that users, regardless of their technical background, can navigate the system with ease. A user-friendly experience minimizes training time and allows for quicker adoption, which is vital in the fast-paced world of finance.
Moreover, scalability is another prominent characteristic. As businesses grow, their data requirements often evolve. MDM solutions that expand easily to accommodate more data without compromising performance are invaluable. The software should ideally include a robust data governance module. This would enable organizations to enforce data policies and ensure compliance across different jurisdictions, offering assurances that data is accurate and trustworthy.
One unique feature of many MDM systems is a centralized data repository. This facilitates a single version of the truth, reducing the chances of discrepancies across departments. However, it's worth noting that while such a feature streamlines data access, it might necessitate significant upfront investment and thorough planning during implementation.
Integration Capabilities
Integration capabilities of MDM software are crucial. They determine how well the MDM system can connect with existing data sources and applications within an organization. An effective MDM solution offers seamless integration with Customer Relationship Management (CRM) and Enterprise Resource Planning (ERP) systems. This ensures data flows smoothly and consistently across platforms, which is a primary goal in master data management.
Another highlight in this area is the software’s support for various data formats and protocols. Being versatile in this aspect allows organizations to merge disparate systems into a coherent data environment. A solution that excels in integration can significantly reduce the time and effort spent on data extraction and preparation for analytical purposes.
On the downside, advanced integration features can complicate initial setups and may require specialized expertise to execute correctly. Organizations might find themselves facing a sizable learning curve.
Data Analytics Tools
Predictive Analytics
Predictive analytics represents a game-changer in finance. By utilizing statistical algorithms and machine learning techniques, organizations can analyze historical data to predict future outcomes. This capability is vital for strategic planning, risk management, and identifying trends that inform decision-making.
A standout feature of predictive analytics is its ability to process large volumes of data efficiently. Consequently, organizations can derive actionable insights much quicker than traditional methods would allow. The predictive models can alert businesses to patterns that might signify a financial downturn or highlight opportunities for investment, thus allowing timely intervention.
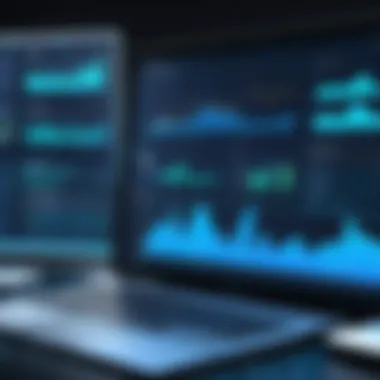
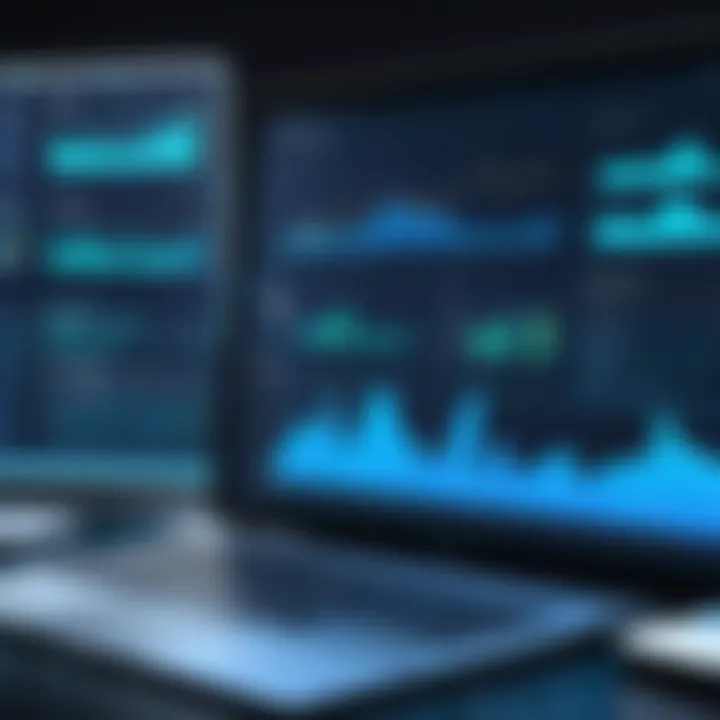
However, the successful implementation of predictive analytics requires high-quality data input. If the data is flawed or inconsistent from the get-go, the output will also be unreliable. Hence, firms must prioritize data cleansing processes before diving into advanced analytics.
Business Intelligence Applications
Business Intelligence (BI) applications have become indispensable for organizations aiming to make data-driven decisions. These tools consolidate high volumes of data from numerous sources and present it in an easily digestible format, such as dashboards and reports.
A key characteristic of BI applications is their ability to enhance visibility across operations. By offering real-time data access, businesses can monitor their financial health and operational metrics effectively. This immediacy is often pivotal when assessing performance against key performance indicators (KPIs).
What sets many business intelligence solutions apart is their interactive visualization capabilities. These features allow users to explore data intuitively, gaining insights from various angles. However, adopting BI applications may warrant substantial investments in training staff to maximize their potential and utilize them efficiently.
In essence, the integration of advanced technological solutions is foundational to successful finance master data management, providing not just structure but also agility in decision-making.
Regulatory Considerations in Finance Master Data
In the intricate world of finance master data management, adhering to regulatory frameworks is not just a checkbox exercise. It’s a critical element that underpins the integrity, reliability, and overall utility of financial data systems. Organizations today operate under intense scrutiny, with regulators setting standards that not only ensure compliance but also foster trust in the financial data that organizations provide to stakeholders. This section sheds light on two vital components of regulatory considerations: compliance requirements and the impact of data privacy laws.
Compliance Requirements
GAAP
Generally Accepted Accounting Principles (GAAP) serve as a cornerstone for financial reporting in the United States. One specific aspect of GAAP is its focus on consistency and transparency in financial statements. By requiring organizations to use standardized accounting methods, GAAP helps maintain a uniform approach to financial reporting, which is essential for comparability across different entities.
The key characteristic of GAAP is its prescriptive nature; it outlines specific rules and guidelines that organizations must follow. This is beneficial for firms as it provides a clear road map for compliance, minimizing the risks of misreporting and enhancing stakeholders' confidence in financial statements.
A unique feature of GAAP is its emphasis on historical cost, which insists on recording assets at their original purchase price. While this offers a more stable perspective on asset values, it can sometimes be seen as a disadvantage, particularly in times of significant market fluctuation where current market values may differ drastically from recorded values.
IFRS
International Financial Reporting Standards (IFRS), on the other hand, is an internationally recognized set of accounting standards that aim to bring transparency, accountability, and efficiency to financial markets worldwide. One crucial aspect of IFRS is its principle-based approach rather than a rule-based set of guidelines, offering more flexibility in how financial reports are prepared.
The key characteristic of IFRS is its adaptability, which is particularly appealing for companies engaged in international business. This adaptability allows firms to present financial statements that better represent their financial position and performance.
However, a unique feature of IFRS is its comprehensive requirement for fair value measurement, which can pose challenges regarding volatility in asset valuations. This can lead to swings in financial statements based on market conditions, which might be seen as both an advantage for transparency and a disadvantage for financial stability.
Impact of Data Privacy Laws
GDPR
The General Data Protection Regulation (GDPR) has dramatically reshaped how businesses manage personal data in the European Union. A specific aspect of GDPR that cannot be overlooked is the focus on consent. Organizations now need to secure explicit consent from individuals before processing their personal data, which encourages greater accountability and transparency.
A key characteristic of GDPR is the right it grants individuals to access their data and request its deletion. This provision enhances trust between organizations and consumers, but it also demands rigorous data management systems that can quickly respond to such requests. The unique feature of GDPR is its extended reach beyond EU borders, requiring any entity dealing with EU citizens' data to comply. While this might seem inconvenient, it fosters a global standard for data protection, benefiting data privacy overall.
CCPA
The California Consumer Privacy Act (CCPA) represents another significant shift in how companies handle personal data. A specific aspect of CCPA is its emphasis on consumer rights, giving individuals control over their personal information and the choice to opt-out of data collection.
The key characteristic of CCPA is its expansive definition of personal information, which includes almost any data that can be linked to an individual. This creates a robust framework for consumer protection and places the onus on businesses to establish clear data management practices. A unique feature of CCPA is the possibility of monetary penalties for non-compliance, which serves as a strong incentive for organizations to prioritize data privacy. However, the constant change in regulations can create challenges for companies trying to keep their data practices aligned.
The blend of compliance and privacy regulations paves the way for a more structured and secure approach to finance master data management, ensuring organizations not only survive regulatory scrutiny but thrive in it.
Future Trends in Finance Master Data Management
In an ever-evolving financial landscape, understanding the future trends in finance master data management is not just beneficial; it is essential. As technological advances reshape how organizations handle data, staying abreast of these changes can enhance decision-making processes and operational efficiency. Various trends are emerging that showcase the crucial role of AI, machine learning, and cloud technologies in streamlining data management.
Artificial Intelligence and Machine Learning
Automated Data Processing
Automated data processing represents a significant leap forward in handling finance master data. This technology allows for increased efficiency by automating repetitive tasks, such as data entry and validation. Organizations benefit from fewer human errors and accelerated processing speeds. One key characteristic of automated data processing is its ability to integrate seamlessly with existing financial systems, making it a favorite among finance professionals looking to optimize workflows.
The unique feature of automated data processing lies in its ability to learn from historical data, refining procedures over time. This can lead to improved data integrity, as the systems identify and rectify discrepancies autonomously. However, reliance on automated systems isn't without challenges; initial setup costs can be high, and organizations must ensure adequate workforce training to adapt to this new paradigm. By embracing automated processing, companies can harness efficiency and focus more on strategic decision-making rather than mundane tasks.
Advanced Predictive Capabilities
Advanced predictive capabilities, fueled by AI and machine learning technologies, offer organizations a chance to anticipate market changes and financial risks with exceptional precision. The predictive models utilize vast datasets, analyzing trends and patterns to deliver actionable insights. This ability is paramount, especially in a financial environment where timely decisions can lead to substantial gains or losses.
Among its many benefits, one key aspect of advanced predictive capabilities is risk assessment. Companies can evaluate investment opportunities and forecast future cash flows, helping mitigate potential pitfalls. However, these systems require accurate input data; otherwise, predictions may lead to misguided strategic choices. The advanced capabilities empower businesses to stay ahead, turning potential challenges into opportunities when managed well.
Cloud Technology Advancements
Flexibility and Scalability
Cloud technology has revolutionized how organizations manage their finance master data through its inherent flexibility and scalability. Using cloud platforms, companies can rapidly adjust their data management capabilities based on their current needs or anticipated growth. This characteristic makes cloud solutions particularly appealing for dynamic finance environments, where changing regulations or market conditions can impact operations significantly.
The unique aspect of cloud solutions is that they offer pay-as-you-go pricing, allowing businesses to optimize their spending. However, organizations must evaluate data security protocols carefully when moving sensitive financial information to the cloud. Flexibility and scalability through cloud advancements can drive efficiency but come with responsibility and vigilance regarding data protection.
Enhanced Collaboration Tools
Enhanced collaboration tools found in cloud environments are integral for finance teams striving for seamless communication and productivity. These tools enable multiple stakeholders to access and modify financial data simultaneously from various locations. The ability to collaborate in real-time diminishes delays and fosters teamwork, leading to well-informed decisions.
One major benefit of enhanced collaboration tools is the breaking down of silos within the organization, ensuring a cohesive approach to finance management. Although these tools increase operational efficiency, challenges such as managing access rights and ensuring data integrity in collaborative environments remain. Nonetheless, investing in appropriate collaboration tools can significantly augment an organization’s data management capabilities, fostering a culture of teamwork across departments.
"The ability to align finance master data management with cutting-edge technologies like AI and cloud solutions not only boosts efficiency but also positions organizations for future growth."