Understanding Big Data Tools: Comprehensive Guide
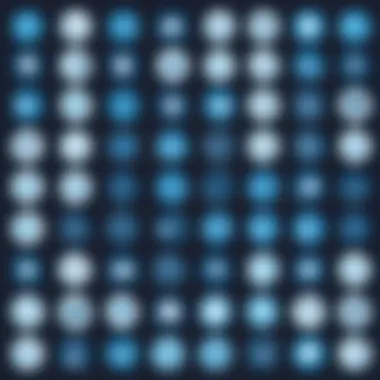
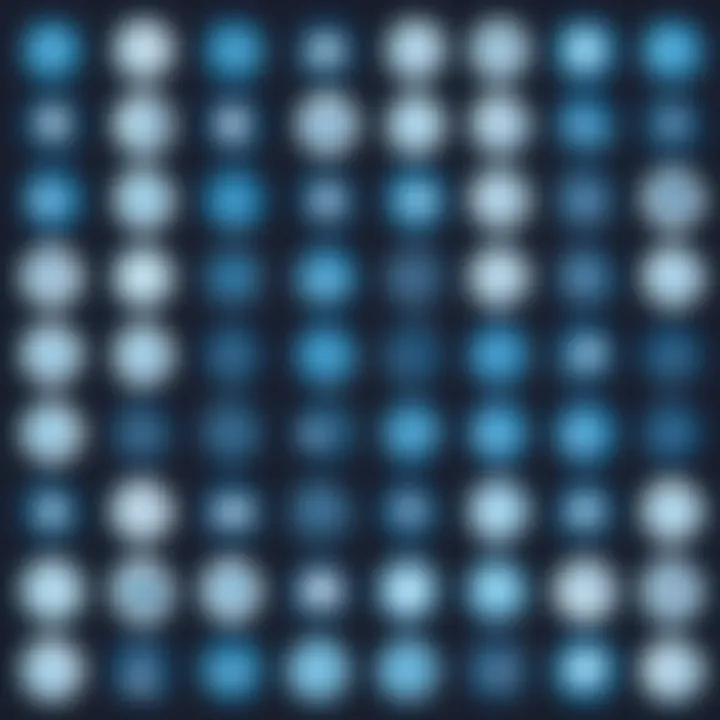
Intro
Big data tools play a vital role in today's data-driven society. As organizations collect massive volumes of data, the need for effective analysis becomes imperative. This article serves as a guide to understanding the various tools available for handling big data. By exploring their functionalities, types, and significance, we aim to empower businesses and IT professionals to make informed decisions.
Understanding how these tools work and their applications can open new pathways for insights and operational efficiencies. From analytics platforms that simplify complex data sets to robust data management solutions, the landscape encompasses a wide range of options.
Furthermore, the focus will be on ensuring readers comprehend key features, how to evaluate them, and the trends emerging in this field.
Software Category Overview
Purpose and Importance
Big data tools are essential for data management and analysis. They help organizations turn raw data into actionable insights. In an era of digital transformation, the importance of these tools cannot be overstated. They allow companies to make data-driven decisions that enhance efficiency, improve customer experiences, and ultimately generate better financial performance.
Some of the core purposes of big data tools include:
- Data Collection: Gather data from various sources seamlessly.
- Data Storage: Store vast amounts of data securely and accessibly.
- Data Processing: Process complex datasets quickly and efficiently.
- Data Analysis: Analyze data for trends, insights, and patterns relevant to business objectives.
Incorporating these tools leads to better resource management and decision-making.
Current Trends in the Software Category
Several trends are shaping the future of big data tools today. One notable trend is the rise of cloud-based solutions. These solutions offer scalability and flexibility, making it easier for organizations to adapt to changing needs. Furthermore, the integration of artificial intelligence and machine learning into data tools is transforming the way businesses analyze data. It enhances predictive analytics capabilities, allowing companies to preemptively respond to market changes.
Moreover, data compliance has gained significant attention. Stricter regulations require tools that not only manage data but also ensure privacy and security. Tools that are built with these considerations are starting to dominate the market landscape.
"The ability to analyze vast amounts of data at unprecedented speeds will define the next decade of business success."
Data-Driven Analysis
Metrics and Criteria for Evaluation
When evaluating big data tools, various metrics must be considered. This ensures the right fit for specific organizational needs. Important criteria include:
- Scalability: Can the tool handle increasing data volumes without compromising performance?
- Usability: Is the interface user-friendly for all levels of technical expertise?
- Integration Capabilities: How well does the tool connect with existing systems?
- Cost Efficiency: Does the pricing model align with the expected return on investment?
By understanding these metrics, organizations can better assess which tools will provide the most value.
Comparative Data on Leading Software Solutions
It's helpful to look at some leading software solutions in the market. For instance, tools like Apache Hadoop, Microsoft Azure, and Tableau have distinct functionalities and strengths that cater to different business needs. Each of these platforms offers unique features designed for data analysis and management, providing companies with choices that align with their specific objectives.
For instance:
- Apache Hadoop is known for its ability to process large datasets reliably.
- Microsoft Azure provides cloud flexibility and integration across various applications.
- Tableau excels in data visualization and intuitive user interfaces.
Evaluating these solutions based on the metrics discussed will yield a comprehensive framework for selecting the most suitable big data tool.
Prolusion to Big Data
In todayβs data-driven world, understanding big data is essential for professionals across various industries. Big data refers to the immense volume of structured and unstructured data generated from various sources. It can come from social media interactions, sensor data, internet of things (IoT) devices, and much more. The sheer size and complexity of this data present both challenges and opportunities for businesses.
By grasping what big data entails, organizations can harness its power to improve decision-making processes, identify emerging trends, and enhance the customer experience. The growing reliance on data has transformed business strategies. Companies that successfully implement big data tools benefit from operational efficiencies and a competitive edge in their market.
Definition of Big Data
Big data can be defined by its three primary characteristics, often referred to as the three Vs: Volume, Velocity, and Variety.
- Volume: This refers to the vast quantities of data generated every second. From millions of tweets to billions of transactions, the amount of data can overwhelm traditional data processing tools.
- Velocity: This indicates the speed at which data is created and processed. Real-time data streaming demands tools that can handle information exchange swiftly and efficiently.
- Variety: Big data comes in various formats, including structured data like databases, semi-structured data like XML, and unstructured data like texts and images. Each format poses unique processing challenges.
This definition highlights the gravity of big data. It is not only about size but about managing various data types quickly to derive actionable insights.
Importance of Big Data in Modern Business
The role of big data in modern business cannot be overstated. Firms leveraging big data enjoy numerous advantages that allow them to thrive in a competitive landscape. Some key benefits include:
- Data-Driven Decision Making: Businesses can use data analytics to make informed decisions. This transformation from intuition-based to data-driven decision-making increases accuracy and effectiveness.
- Enhanced Customer Insights: Organizations can gather and analyze customer behavior data. Understanding preferences helps create personalized marketing strategies that improve customer engagement.
- Operational Efficiency: Big data analytics reveal inefficiencies within processes. Firms can identify bottlenecks and optimize resources to improve overall organizational performance.
- Risk Management: Predictive analytics enables businesses to foresee potential risks and implement strategies to mitigate them. Understanding market trends can assist in forecasting changes, thus preparing organizations.
Understanding Big Data Tools
Big data tools represent a critical backbone for organizations managing massive volumes of information today. They facilitate the process of collecting, storing, processing, and analyzing data efficiently. As businesses increasingly rely on data-driven decisions, understanding these tools becomes essential for professionals aiming to leverage data for strategic advantages. The nature of big data demands specialized tools capable of handling its exponential growth and complexity, thus, comprehension of these tools is not merely beneficial but necessary.
This article aims to delineate the attributes and functionalities of big data tools, offering insights that empower businesses to navigate this convoluted landscape. By understanding the capabilities and limitations of various tools, businesses can make informed choices aligning with their organizational strategies and operational requirements.
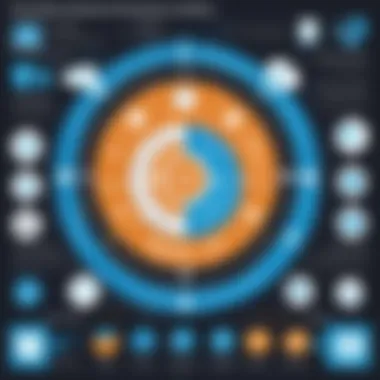
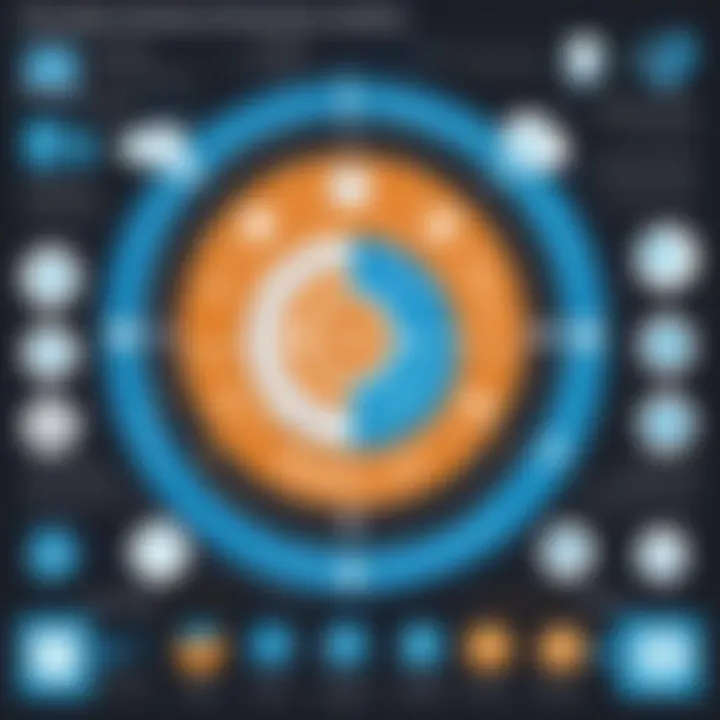
What Constitutes a Big Data Tool
A big data tool is defined as software or a platform that facilitates the management, processing, and analysis of large datasets. Such tools cater specifically to the demands of big data characteristics: volume, velocity, and variety. Each tool may serve different purposes in the data lifecycle, falling under several categories like data storage, processing, and visualization.
Key examples of big data tools include:
- Hadoop: A framework that allows for the distributed processing of large datasets.
- Apache Spark: Offers rapid processing of data in-memory, making it efficient for both batch and real-time analytics.
- NoSQL databases: Such as MongoDB or Cassandra, designed to manage unstructured data.
These tools play a vital role in enabling organizations to collect and analyze data from multiple sources, thus providing a more comprehensive view of their operations and customer behavior.
Key Features of Big Data Tools
When selecting big data tools, certain key features should be considered to ensure they meet the necessary requirements of a business. Some of the most critical features include:
- Scalability: The ability to grow and handle increasing data loads without significant performance degradation.
- Flexibility: Support for various data types, including structured, semi-structured, and unstructured data, ensuring compatibility with diverse data sources.
- Real-time Processing: Allows organizations to make immediate insights from data as it is generated, crucial for time-sensitive decision-making.
- Robust Security: As data sensitivity is paramount, tools must incorporate features that ensure data protection and compliance with regulations.
- User-Friendly Interface: A straightforward interface enables professionals across different disciplines to understand and utilize the tool effectively, without requiring extensive training.
Understanding these features enables organizations to pinpoint the specific tools that align with their operational goals and data strategy.
Categories of Big Data Tools
The landscape of big data is vast and complex, demanding diverse approaches to handle, process, and analyze the massive volumes of information generated daily. Understanding the various categories of big data tools is crucial for organizations looking to leverage data for strategic advantage. Each category serves a specific purpose and addresses unique challenges, making it imperative for businesses to choose the right tools tailored to their requirements.
Data Storage Solutions
Data storage solutions are foundational to any big data strategy. These tools enable the efficient capturing, storing, and managing of large datasets. In a world where data is expanding exponentially, having robust storage solutions is not just important; it is essential. Traditional databases can struggle under the weight of unstructured or semi-structured data, such as social media interactions or sensor data from IoT devices. This scenario creates a pressing need for specialized storage systems designed for big data.
There are several types of data storage solutions available:
- Hadoop Distributed File System (HDFS): A popular choice for scalable storage. It divides data into smaller blocks and distributes them across a cluster, ensuring fault tolerance.
- NoSQL databases: Solutions like MongoDB and Cassandra provide flexible schema designs that can accommodate various data types and structures, making them ideal for big data environments.
- Cloud storage solutions: Services like Amazon S3 and Google Cloud Storage offer scalable, on-demand data storage, eliminating the need for extensive physical hardware.
Choosing the appropriate data storage solution involves evaluating factors such as scalability, speed, and the type of data being processed. Organizations must also consider their long-term data growth and access requirements.
Data Processing Tools
Data processing tools play a pivotal role in extracting insights from raw data. These tools are designed to handle large volumes of information quickly and effectively. With the right processing tools, businesses can perform advanced analytics, automate data workflows, and derive value from their data assets.
Key categories of data processing tools include:
- Batch Processing Tools: Platforms like Apache Hadoop enable the processing of large datasets in batches, making it suitable for complex calculations and historical data analysis.
- Streams Processing Tools: Apache Kafka and Apache Flink specialize in real-time data processing, allowing organizations to analyze data as it arrives and respond swiftly to events.
- ETL (Extract, Transform, Load) Tools: Tools such as Talend and Apache NiFi automate data integration processes, aiding in data cleaning, transformation, and movement.
Additionally, flexibility and performance should be key considerations when selecting data processing tools. Organizations need to assess how well these tools can handle their specific data types and processing demands.
Data Visualization Tools
Data visualization tools are crucial for transforming raw data into meaningful insights that can drive decision-making. They enable users to create graphical representations of data, making complex information more accessible and understandable. An effective visualization tool can bridge the gap between data analysts and business stakeholders, fostering a shared understanding of data-driven insights.
Popular data visualization tools include:
- Tableau: Known for its ease of use and powerful visualization capabilities, allowing users to create interactive dashboards effortlessly.
- Power BI: A Microsoft product that integrates well with other Microsoft services, providing a user-friendly platform for business intelligence.
- D3.js: A JavaScript library for producing dynamic and interactive visualizations in web browsers.
Organizations should focus on usability, integration capabilities, and the ability to handle large datasets when evaluating visualization tools. Tailoring these tools to the audience's needs is equally important to ensure effective communication of insights.
"Choosing the right category of big data tools is critical for maximizing data value and ensuring scalability as data demands continue to evolve."
Understanding the categories of big data tools enables organizations to develop a cohesive strategy for managing and utilizing their data effectively. In the next sections, we will explore popular big data tools in greater detail, focusing on their functionalities and best use cases.
Popular Big Data Tools
The category of popular big data tools is crucial in today's data-driven landscape. These tools handle vast amounts of data efficiently and effectively, enabling businesses to glean meaningful insights. The right big data tool can streamline processes, reduce costs, and improve decision-making capabilities.
Overview of Hadoop and Its Ecosystem
Hadoop is a widely recognized framework that allows for distributed storage and processing of large datasets across clusters of computers. An important aspect is its ability to scale from a single server to thousands of machines. This flexibility is key for handling big data, as data grows exponentially.
Hadoop's Ecosystem encompasses several components, including:
- Hadoop Distributed File System (HDFS): This storage system enables high-throughput access to application data.
- MapReduce: This programming model processes data in a distributed manner, dividing tasks into manageable pieces.
- Apache Hive: This data warehouse software is built on top of Hadoop, allowing for SQL-like queries on the dataset.
The ecosystem makes Hadoop versatile for various industries, enhancing its adoption among organizations looking to leverage big data analytics.
Intro to Apache Spark
Apache Spark is another leading tool in the big data ecosystem, known for its speed and ease of use. Unlike Hadoop's MapReduce, Spark performs in-memory data processing. This feature significantly boosts the performance of big data analytics tasks.
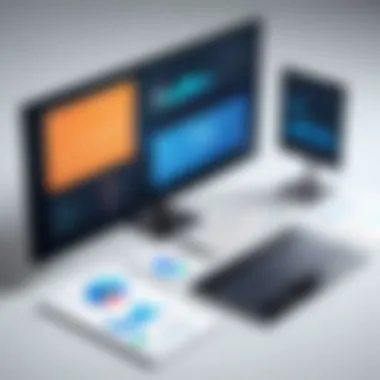
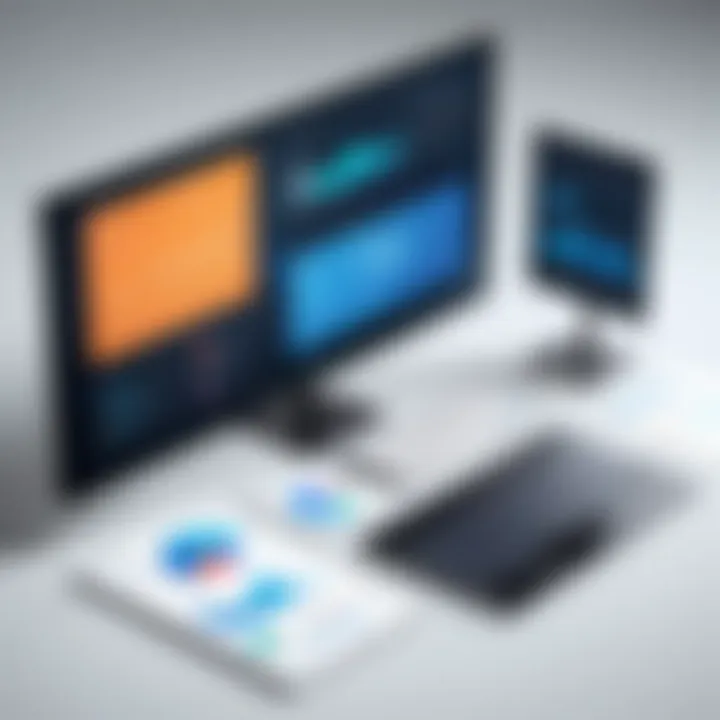
Key features of Apache Spark include:
- Fast Processing: The capability to handle real-time data streams.
- Ease of Use: Offers APIs in various programming languages including Java, Scala, and Python.
- Advanced Analytics: Supports advanced analytics such as machine learning and graph processing.
Organizations favor Spark when quick insights are required from their data due to its efficiency and user-friendly design.
Exploring NoSQL Databases
NoSQL databases represent a shift from traditional relational databases. They are designed to handle unstructured or semi-structured data, accommodating the needs of big data applications.
Key characteristics of NoSQL databases include:
- Scalability: They support horizontal scaling, allowing businesses to add more servers easily.
- Flexibility: NoSQL can accommodate various data models such as document, key-value, and graph databases.
- High Availability: They are often designed to provide high availability and fault tolerance.
Examples of popular NoSQL databases include MongoDB, Cassandra, and Couchbase. Each has distinctive features that serve different business requirements.
By using these tools, businesses can gain competitive advantages through innovative data-driven strategies.
Big Data Analytics
Big Data Analytics plays a crucial role in extracting value from large datasets. In an era where data is often referred to as the new oil, the ability to effectively analyze vast streams of information becomes essential for competitive advantage. Businesses leverage analytics to form insights that inform strategic decisions. Understanding the nuances of Big Data Analytics empowers firms to uncover hidden patterns, identify trends, and ultimately enhance decision-making processes.
Data analytics can be categorized into various types that serve diverse purposes depending on business needs. The types of analytics vary in complexity, from simple descriptive analytics to more sophisticated prescriptive analytics. Each type presents unique benefits, which organizations can harness to address specific problems, improve operational efficiency, or enhance customer engagement.
In addition to understanding the types of analytics, businesses also benefit from using various analytic tools available on the market. These tools help organizations in processing these large datasets efficiently and accurately, thus minimizing errors and ensuring insightful conclusions.
Overall, Big Data Analytics is not just a technological solution; it embodies a transformative approach that allows organizations to adapt in a continuously evolving digital landscape. The significant return on investment from leveraging analytics justifies its increasing incorporation into various business processes.
Types of Analytics in Big Data
- Descriptive Analytics: This type focuses on summarizing historical data to identify trends and patterns. Tools used include dashboards and reporting software, which visualize data in an understandable format.
- Diagnostic Analytics: This goes a step further by analyzing past performance to understand why certain outcomes occurred. Techniques such as data mining and complex event processing are common to interpret these insights.
- Predictive Analytics: Predictive analytics uses statistical models and machine learning techniques to forecast future events based on historical data. The effectiveness in predicting consumer behavior makes this type popular among marketing teams.
- Prescriptive Analytics: This type recommends actions to achieve desired outcomes. It often involves optimization and simulation algorithms to provide actionable insights. Prescriptive analytics is increasingly valuable for supply chain management and decision-making.
- Real-Time Analytics: This enables organizations to analyze data as it is generated. This type is crucial for businesses that need to respond swiftly to live data changes, such as financial trading or fraud detection.
Tools for Data Analytics
Selecting the right tools for data analytics is critical for operational success. There are various tools available, each serving different functions. Here are some of the prominent tools:
- Tableau: This is a powerful visualization tool that allows users to create interactive dashboards to analyze business data.
- Apache Hadoop: It provides a framework for distributed storage and processing of large datasets across clusters of computers.
- Google Analytics: Mainly used for web analytics, this tool helps businesses track and report website traffic, allowing them to enhance their online presence.
- IBM SPSS: A robust tool for statistical analysis, it is designed for data mining, text analytics, and statistical analysis.
Emerging tools continue to evolve, offering new functionalities tailored for big data environments. Adopting the correct tool aids organizations in maximizing their analytics potentials and making data-driven decisions effectively.
"The future of business lies in those who can effectively harness and leverage the insights derived from their data."
Evaluating Big Data Tools
In the rapidly evolving landscape of big data, selecting the right tools is paramount for success. Organizations face a myriad of choices that can have a significant impact on their data strategy. Evaluating big data tools entails understanding not just their functionalities, but also their fit within specific organizational needs. The effectiveness of data-driven decisions hinges on choosing tools that align effectively with business objectives.
The evaluation process involves several key considerations, including scalability, user-friendliness, integration capabilities, and support services. Companies need a solution that grows with their data needs without incurring excessive costs or complexity. A careful assessment leads to increased productivity and strategic advantages.
Furthermore, proper evaluation reduces the risk of investing in inadequate tools. Tools that do not meet expectations can lead to wasted resources and hinder data quality. This section unpacks the criteria for selection and cost considerations.
Criteria for Selection
When considering big data tools, several selection criteria should be at the forefront.
- Scalability: Can the tool handle increasing amounts of data as your business expands? A scalable solution is crucial for growing organizations.
- Integration: Assess how well the tool integrates with existing systems. Poor integration can lead to bottlenecks in workflow.
- Ease of Use: Tools should be intuitive for both technical and non-technical users to ensure widespread adoption.
- Support and Community: Evaluate the availability of vendor support and the community surrounding the tool. A robust community can provide valuable resources and knowledge.
- Performance and Speed: The tool must deliver fast processing times to handle complex data analytics tasks effectively.
Through careful analysis of these criteria, organizations can find tools that not only fit their current needs but also anticipate future growth.
Cost Considerations
Budgeting for big data tools is a critical aspect of the evaluation process.
When weighing costs, businesses must go beyond the initial purchase price. Consider the following factors:
- Upfront Costs vs. Long-term Investment: Some tools may appear cheaper initially but come with higher operating costs down the line.
- License Fees: Understand the pricing model, whether it's subscription-based, per user, or a flat fee. Each model impacts budgeting differently.
- Hidden Costs: Investigate potential hidden costs such as training, maintenance, or upgrades. These can accumulate and affect overall expenses.
- ROI Analysis: Consider the return on investment potential. A higher quality tool may require a more significant upfront cost, but can lead to greater efficiencies and savings over time.
"The right big data tool is not just a purchase; it is an investment in your organization's future data capabilities."
Engaging in diligent evaluation ensures that resources are allocated effectively, paving the way for successful data management and analytics.
Implementation Challenges
In the realm of big data, organizations face several implementation challenges that can hinder the effective utilization of tools and technologies. Understanding these obstacles is crucial for businesses aiming to leverage big data for improved decision-making and operational efficiency. This section outlines the significance of implementation challenges, emphasizing specific elements and considerations involved in navigating these hurdles.
Technical Barriers
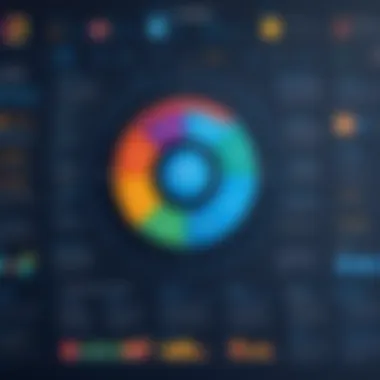
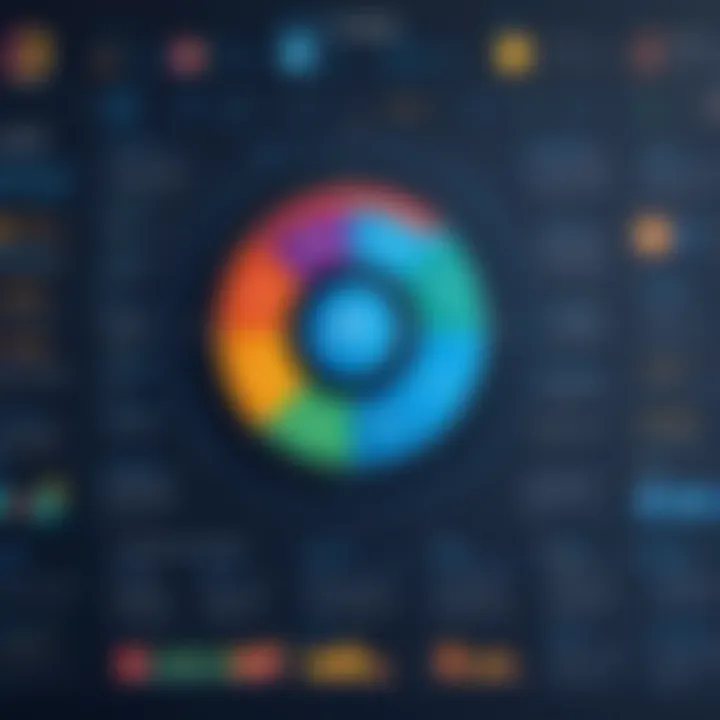
Technical barriers represent one of the foremost challenges in implementing big data tools. They often stem from the complexity of various technologies and the need for specialized knowledge. Organizations must contend with a variety of programming languages, frameworks, and architectures, which can create confusion and slow down implementation.
- For instance, a company integrating Apache Hadoop may encounter issues related to cluster management and data ingestion. These technical complexities often necessitate extensive training for IT staff, elevating both time and resource expenditures.
- Additionally, compatibility issues between existing systems and new tools can complicate implementation efforts. Organizations may find that their legacy systems lack flexibility, making it difficult to integrate big data solutions. This reinforces the necessity for a thorough assessment of existing infrastructure prior to the selection of big data tools.
Overcoming technical barriers requires a strategic approach. It is beneficial to establish a skilled team with expertise in big data technologies, who can provide insights into the appropriate tools and configurations needed for successful implementation. Regular team training and workshops can help bridge knowledge gaps, facilitating a smoother transition to advanced big data applications.
Data Quality Issues
Data quality issues are another prominent challenge organizations should address during the implementation of big data tools. Poor data quality can undermine the integrity of analytical outcomes, resulting in misguided business decisions. Ensuring high-quality data is essential, as it increases the reliability of insights derived from big data analytics.
- There are several factors that contribute to data quality issues, including data inconsistencies, missing values, and inaccuracies. For example, inconsistent data formats from various sources can impede effective analysis and lead to incomplete insights. Consequently, organizations must invest in data cleansing and validation processes to enhance data quality.
- Furthermore, insufficient metadata management can obscure the context and meaning of large datasets. Without proper metadata, it can be challenging to ascertain data origins, transformations, and usage history. This complicates the task of assessing whether data is fit for analytical purposes.
To mitigate data quality issues, organizations should adopt rigorous data governance practices. Establishing guidelines for data collection, maintenance, and quality assurance can significantly improve overall data reliability. Additionally, implementing automated data monitoring tools can assist in quickly identifying and addressing data quality problems.
"Quality data is the foundation of effective big data analytics; without it, the transformative potential of big data tools is severely limited."
Case Studies of Big Data Implementation
Examining case studies of big data implementation is crucial to understanding the real-world application and benefits of these tools. These case studies provide tangible evidence of how businesses leverage big data to enhance decision-making, optimize processes, and drive growth. They also illustrate the variety of use cases, ranging from healthcare to finance, highlighting the versatility of big data tools across different industries.
Big data tools enable organizations to analyze complex datasets, derive insights, and foster data-driven cultures. Insights from case studies reflect both successful strategies and potential pitfalls, serving as a guide for companies considering similar paths. Learning from successes and failures aids in refining approaches and improving future implementations.
Success Stories
Several organizations have set commendable examples through successful big data implementations. One notable case is Netflix. The streaming giant utilizes big data analytics comprehensively to understand viewer preferences and behavior. By analyzing vast amounts of data on viewing patterns, Netflix is able to recommend shows tailored to individual user preferences, improving engagement and retention.
Another success story comes from Walmart, which employs big data to optimize its supply chain. By analyzing customer purchasing trends, they adjust inventory levels and streamline logistics. This data-driven approach not only enhances efficiency but also boosts customer satisfaction by ensuring that products are available when and where customers want them.
Some key takeaways from these successes include:
- Data-Driven Decisions: Organizations should prioritize data analytics to inform strategic decisions.
- Customer Understanding: Analyzing customer behavior helps tailor services to meet actual needs.
- Efficiency Gains: Using data to optimize operations can lead to significant cost reductions and improved service delivery.
Lessons Learned from Failures
Not every implementation yields the desired results. Organizations often face challenges that lead to failures. One example is Target, which faced backlash after its data-driven marketing strategy inaccurately targeted customers based on predictive analytics. Their analysis revealed pregnancies before customers were ready to announce them publicly, creating a public relations challenge.
Another cautionary tale involves a startup that invested heavily in a big data project but faced data quality issues. Improper data management led to inaccurate analyses, rendering insights unreliable and causing strategic missteps.
Key lessons from such failures include:
- Quality Over Quantity: Ensuring data accuracy is critical before performing any analyses.
- Understanding the Audience: Companies must gather insights without invading consumer privacy or comfort.
- Iterative Approach: Implement big data solutions gradually and adjust based on feedback and results.
Case studies serve as both an inspiration and a warning. They illustrate the extensive possibilities offered by big data tools while also highlighting the importance of a robust implementation strategy.
Future Trends in Big Data Tools
The landscape of big data tools is rapidly evolving. Understanding future trends is crucial for businesses seeking to leverage these tools effectively. This section discusses emerging technologies and the impact of artificial intelligence. Each of these elements plays a significant role in how tools will shape data management and analytics going forward.
Emerging Technologies
Emerging technologies in big data are driving changes that enhance data processing and analysis. One key area is the rise of cloud computing. Many organizations are moving their data access and storage to the cloud. This transition allows for greater scalability and flexibility. As data grows, cloud solutions can adapt in ways traditional on-premises systems cannot.
Another notable trend is the use of edge computing. With the Internet of Things (IoT), data is generated at the edge of networks, leading to the necessity for tools that process data in real-time. This helps businesses access insights without the latency associated with sending data to centralized systems.
- Data Lakes: Enabling storage of vast amounts of unstructured data, providing a foundation for future analytics.
- Serverless Computing: Reducing the need for server management allows developers to focus on building applications rather than infrastructure.
- Graph Databases: These are emerging as powerful solutions for handling complex relationships within data, offering superior performance for certain analytical workloads.
Impact of Artificial Intelligence
Artificial Intelligence (AI) is fundamentally reshaping big data tools. AI enhances data analysis and predictive modeling, offering more accurate insights. Machine learning algorithms can process large datasets far more efficiently than traditional methods. This allows organizations to uncover patterns and trends that may go unnoticed.
The integration of AI into big data tools accelerates automation in data management. Tools equipped with AI capabilities can automate data cleansing, transformation, and even some decision-making processes. Moreover, this reduces human error and frees up time for data scientists to focus on more strategic tasks.
AI-driven analytics can lead to:
- Real-time Insights: Providing adaptive responses based on current data.
- Personalized Experiences: Tailoring user interactions based on behavior analytics.
- Predictive Maintenance: Utilizing data to foresee potential failures, particularly in industrial applications.
"AI's capability to analyze vast datasets will continue to fuel innovation, making it central to future big data strategies."
In summary, understanding these future trends empowers organizations to select tools that will not only serve their current needs but also adapt and evolve as technology progresses. Engaging with these trends will position businesses to harness the full potential of big data in coming years.
End
In this article, we have explored the multifaceted world of big data tools. Such tools are critical in helping businesses manage, analyze, and visualize large sets of data. The complexity of big data demands specialized tools to extract meaningful insights. As data continues to grow in volume and variety, understanding these tools becomes essential for any organization aiming to leverage data for competitive advantage.
Summary of Key Points
- The definition of big data encompasses large volumes of structured and unstructured data.
- Big data tools play a vital role in data storage, processing, and visualization.
- Businesses must select tools based on specific needs and criteria, including scalability and cost.
- Understanding emerging technologies can help organizations remain adaptable in a rapidly changing environment.
- Successful implementations showcase the benefits of adopting these tools, while failed cases offer valuable lessons.
Final Thoughts on Big Data Tools
Big data tools represent a significant advancement in our ability to make sense of data. They provide businesses with the means to uncover insights that were once hidden in vast datasets. Organizations should consider various factors, including ease of integration and user-friendliness, when selecting the right tools. The conversation around big data does not end with current tools; rather, it extends into the future with advancements in AI and machine learning poised to further enhance data analytics capabilities. As we move forward, staying informed about developments in big data tools is crucial for maintaining a cutting-edge advantage in any industry.