Understanding Chat Sentiment Analysis: Key Insights
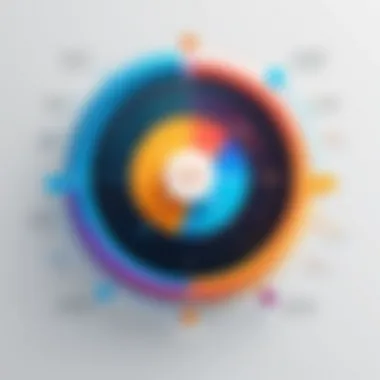
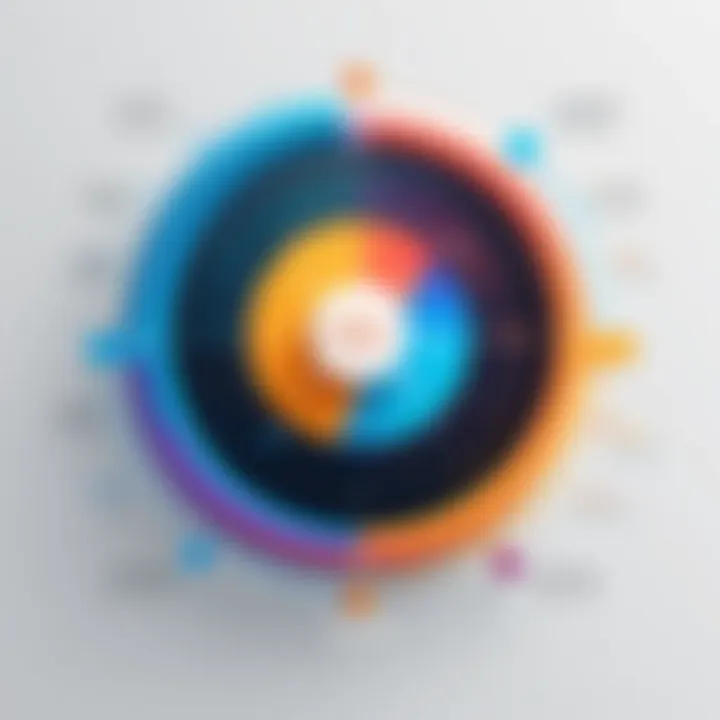
Intro
In a world driven by digital communication, the nuances of human sentiment often get lost amidst the flood of words exchanged in chats. As customer interactions increasingly move to instant messaging platforms, understanding the underlying emotions can profoundly influence business outcomes. Here, we will explore the intricate field of chat sentiment analysis, highlighting its significance and multifaceted applications.
More than just interpreting text, sentiment analysis digs into the realm of human behavior, enabling organizations to tailor their strategies effectively. From customer service representatives gauging user frustration to product developers identifying areas for improvement based on feedback, the capability to analyze sentiment can be a game-changer.
This section serves as a gateway into the core concepts and methodologies that underscore sentiment analysis. By peeling back the layers, we uncover how organizations can harness these insights to foster better communication and derive actionable intelligence. To that end, let's dive into an overview of software in this category and their pivotal roles in contemporary sentiment analysis.
Prelims to Chat Sentiment Analysis
In today's fast-paced digital landscape, understanding the nuances of how people express themselves in chat conversations has become more vital than ever. The concept of chat sentiment analysis emerges as a cornerstone in deciphering these exchanges. This process entails examining textual data to gauge emotional tone, allowing businesses and individuals to draw valuable insights from seemingly mundane interactions. Not just for linguists or tech folks, this analysis has real-world implications, particularly in customer service, marketing, and brand management.
Definition of Sentiment Analysis
At its core, sentiment analysis is about categorizing emotions embedded in text. It helps to determine whether a piece of writing expresses a positive, negative, or neutral sentiment. Think of it as a sophisticated emotional barometer that gauges the atmosphere of communication. Unlike face-to-face chats, where tone and body language provide context, written conversations often lack these cues, ensuring that the role of sentiment analysis becomes even more crucial. Basically, you can spot trends in customer feelings towards your product or service without needing them to fill out a survey.
Importance in Digital Communication
In the realm of digital communication, sentiment analysis holds the torch as a guiding light to understanding customer interactions. Businesses today are inundated with feedback, from reviews to social media comments. The importance here cannot be overstated: it allows companies to adapt their strategies based on the emotional climate surrounding their offerings. By identifying pain points or trending praises, organizations can fine-tune their products and services, enhancing the overall customer journey. Imagine receiving real-time insights about the client's perspective! That's the kind of agility that keeps a company two steps ahead of the competition.
Evolution of Sentiment Analysis Techniques
The field of sentiment analysis has not been stagnant; rather, it’s undergone a significant metamorphosis over the years. In the early days, sentiment analysis relied heavily on simple keyword recognition and manual coding, which wasn’t reliable and often led to skewed interpretations. You could say it was akin to painting by numbers without the full picture.
Fast forward to today, and we have advanced algorithms powered by natural language processing that analyze text on a much deeper level. Machine learning models can now recognize context and subtleties in language, greatly enhancing the accuracy of the analysis. This progression indicates a large shift from basic counting of 'likes' or 'dislikes' towards a fuller understanding of what sentiments actually convey.
"The right sentiment analysis tools can empower your business decisions, allowing for more meaningful interactions with customers.”
In summation, the introduction to chat sentiment analysis is not merely an academic exercise. It encapsulates a practice that can fundamentally alter how organizations approach their customer interactions, allowing for a more responsive and empathetic dialogue. As the digital landscape continues to evolve, staying updated with tools and techniques in this arena will be paramount for those aiming to excel.
How Sentiment Analysis Works
Understanding how sentiment analysis operates is central to grasping its effectiveness in processing and interpreting human emotions in text. This section outlines the vital components and methodologies that give this technology power. The nature of chat interactions requires nuanced comprehension, and sentiment analysis allows businesses to tap into customer sentiments like never before.
Data Collection and Preparation
Before any analysis can take place, data collection and preparation are fundamental steps. You can't build a house without solid bricks. Here’s a closer look:
- Data Sources: Organizations gather data from various platforms—social media, customer service chat logs, online reviews, and even emails. It’s akin to fishing in different ponds for the best catch.
- Data Cleaning: Raw data often contains noise—unwanted characters, irrelevant information, or inconsistent formats. Cleaning this data is like polishing a rough diamond to bring out its brilliance. That means removing duplicates, correcting typos, and standardizing formats.
- Annotation: Once cleaned, a subset of data is particularly annotated with sentiment labels, such as positive, negative, or neutral. This labeled data serves as a training set for subsequent analysis.
- Storage and Management: Storing this data demands suitable management strategies; think of it as organizing a toolbox. You need to know where your tools are to use them effectively.
Effective data collection and preparation are the bedrock upon which robust sentiment analysis is built. Missing steps here could mean the difference between accurate insights and a jumbled mess of data.
Natural Language Processing (NLP) Techniques
Natural Language Processing, or NLP, is the bridge between human language and computer understanding. With algorithms designed to help machines decipher human feelings expressed in text, NLP is essential in sentiment analysis. Here are key techniques:
- Tokenization: The first step in NLP involves breaking down sentences into individual words or phrases, called tokens. This helps in understanding the structure and meaning of the text.
- Sentiment Lexicons: Using dictionaries that map words or phrases to their emotional value is common. These lexicons allow systems to determine sentiment based on predefined emotional weights. For instance, words like "fantastic" might carry a more significant positive weight than "good."
- Part-of-Speech Tagging: By identifying the function of each word in a sentence (noun, verb, adjective, etc.), sentiment analysis can gain better context. Understanding nuances is crucial; for example, "not good" signals a negative sentiment despite the presence of "good."
- Dependency Parsing: This technique explores the relationships between words in a sentence. It provides insight into how sentiments might be affected by context, a bit like unraveling the threads that weave a story together.
NLP techniques are vital for ensuring that sentiment analysis goes beyond surface-level understanding and dives into the depths of emotional expression.
Machine Learning Models for Sentiment Classification
The final piece of the sentiment analysis puzzle involves utilizing machine learning models for sentiment classification. These models learn patterns from training data to make predictions about unseen text. Here’s what you need to know:
- Supervised Learning: This model learns from a labeled dataset, permitting it to classify new data accurately. It’s most like teaching a child with flashcards—showing examples until they recognize patterns.
- Unsupervised Learning: This approach uses algorithms to group data without labeled sentiments. While less common for direct sentiment classification, it’s useful in identifying faceless opinions and trends in massive datasets.
- Common Algorithms:
- Model Evaluation and Tuning: Regular evaluations through techniques such as cross-validation help ensure the model's predictions are both accurate and reliable. It’s like fine-tuning an engine for optimal performance.
- Naive Bayes: Assumes independence among predictors, which is fast and effective for simple classification tasks.
- Support Vector Machines (SVM): These build hyperplanes to separate different sentiment classes in a multi-dimensional space.
- Deep Learning: Neural networks excel at sentiment analysis through their ability to learn complex patterns. Models such as recurrent neural networks (RNN) are particularly adept because they can account for the sequence of words, generating a more profound comprehension of language.
Using appropriate machine learning models during the sentiment classification process significantly elevates the accuracy and depth of sentiment analysis, enabling a clearer picture of customer sentiment and nurturing actionable insights.
Types of Sentiment Analysis
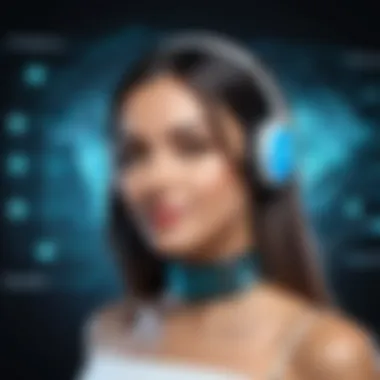
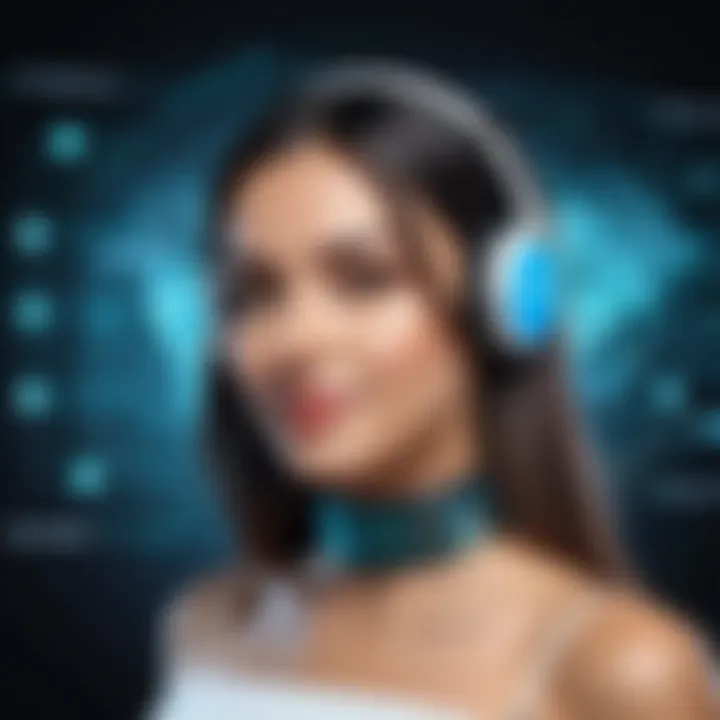
Understanding the different types of sentiment analysis is crucial for grasping how organizations can dissect natural language data to extract meaningful insights. Each method has its merits and drawbacks, thus making it essential to choose the correct approach based on specific goals and contexts. Companies, marketers, and researchers alike benefit from aligning the techniques they use with the intricacies of language and communication.
Rule-Based Sentiment Analysis
Rule-based sentiment analysis utilizes a set of predefined linguistic rules to evaluate sentiment. These rules often consist of lists of words or phrases categorized as positive, negative, or neutral. For instance, an organization might employ a lexicon where words like "happy" and "great" are tagged as positive while words such as "bad" and "sad" are recognized as negative.
One notable advantage of this method is its straightforwardness. It can be implemented without heavy computational resources, which is especially beneficial for smaller projects or organizations that are just dipping their toes in sentiment analysis. However, there are also some limitations to consider:
- Inflexibility: The fixed rules may not adapt well to the nuances and complexities of human language, such as sarcasm or changing connotations of words.
- Oversimplicity: By relying primarily on listed words, the method may miss the overall context, leading to misleading sentiment assessments.
Machine Learning-Based Approaches
Machine learning approaches to sentiment analysis build on the limitations of rule-based methods by leveraging algorithms that learn from data. These models can be trained using labeled datasets—examples of text with predefined sentiments. By processing these datasets, the models recognize patterns and can generalize to classify new, unseen data effectively.
Benefits of this approach include:
- Flexibility: Models can adapt to new linguistic trends and evolving language use.
- Accuracy: Generally, machine learning techniques yield higher precision rates, especially when working with large datasets.
However, these methods are not without downsides. They can require extensive amounts of data for training and may demand significant processing power and resources. Moreover, the performance is heavily reliant on the quality of data fed into the models.
Deep Learning Techniques
Deep learning represents the next frontier in sentiment analysis. This method employs neural networks, particularly those architectures designed to process vast datasets—think of convolutional neural networks and recurrent neural networks. Deep learning can capture intricate patterns in the data, offering nuanced insights into sentiment.
Some relevant perks include:
- Feature Extraction: Deep learning models automatically perform feature extraction, allowing them to identify important variables without needing manual rules.
- Contextual Understanding: These techniques can better understand context and complex sentence structures, capturing sentiment hidden in subtleties.
Still, deep learning isn't a panacea. The models often require considerable computational resources, and their complexity makes them less interpretable than simpler machine learning or rule-based methods.
In sum, the choice between these methods hinges on numerous factors, including project scope, available resources, and desired precision levels. Adapting the chosen approach to the unique nuances of language can offer organizations more profound insights into the emotional undercurrents of chat interactions.
"Choosing the right sentiment analysis technique is like selecting the right tool for a specific job; using the wrong approach can lead to a muddled understanding of user sentiment."
In the ever-evolving landscape of digital communication, understanding these methods lays the groundwork for harnessing the full potential of sentiment analysis.
Applications of Chat Sentiment Analysis
Chat sentiment analysis has emerged as a powerful tool in modern digital landscapes, offering significant insights across various domains. Its applications stretch from customer service to market research, playing a crucial role in shaping business strategies and enhancing communication effectiveness. By analyzing the sentiments expressed in chat conversations, organizations can adapt their approaches to meet user expectations and improve overall engagement. The underlying principle is simple yet profound: understanding the emotions and opinions of users leads to better decision-making and more effective service delivery.
Customer Service Enhancement
In the realm of customer service, the importance of chat sentiment analysis can hardly be overstated. It allows businesses to gauge customer emotions in real time, providing valuable feedback that can refine service strategies. When customers express their feelings—be it frustration, satisfaction, or indifference—analyzing these sentiments helps companies respond appropriately. Implementing sentiment analysis tools can automate this process, ensuring immediate attention to negative sentiments and proactively addressing concerns before they escalate.
- Example: Picture a customer who has been troubleshooting an issue with a product. Through chat support, their sentiment shifts from frustrated to relieved after receiving help. Tracking these emotional changes can inform support teams about the effectiveness of their solutions and allow them to fine-tune their responses.
Additionally, sentiment analysis aids in identifying trends in customer feedback, enabling businesses to adapt their services to better meet client needs. By monitoring sentiment over time, organizations can recognize prevailing issues and make data-driven adjustments, boosting overall satisfaction.
Market Research and Brand Management
Market research has evolved significantly with the introduction of sentiment analysis. Companies leverage sentiment data not just for understanding existing customer attitudes but also for predicting future trends. By examining how consumers feel about different aspects of a brand—be it a marketing campaign, product features, or customer service experiences—businesses gain a competitive edge in the marketplace.
- Application: For instance, a company launching a new product can analyze chat sentiments related to the product’s specifics ahead of its release. If the feedback is largely positive, they may consider highlighting those features in their promotional efforts. Conversely, if the sentiment is negative, adjustments can be made before the product hits the shelves.
Furthermore, brand management benefits from continuous monitoring of online conversations. This not only includes social media but also chats and forums where people discuss products and experiences. By keeping a pulse on consumer sentiment, brands can engage with their audience more effectively, addressing concerns and celebrating positive feedback, thus building a community around their brand.
Social Media Monitoring
Social media platforms have become critical channels for expressing sentiments about brands, products, and services. Chat sentiment analysis can be effectively applied here, allowing organizations to monitor real-time feedback on their social media interactions. Given the fast-paced nature of these platforms, timely sentiment analysis can illuminate public perception, helping brands understand reactions to their campaigns and content.
"Understanding sentiment on social media not only aids in managing brand reputation but also informs content strategy, ensuring that messages resonate with the audience."
Through sentiment analysis, brands can identify influencers and ambassadorship opportunities as well. When a user expresses appreciation or enthusiasm through social media chats or comments, this information can help brands engage with those individuals, fostering deeper relationships and potentially harnessing their influence for marketing purposes.
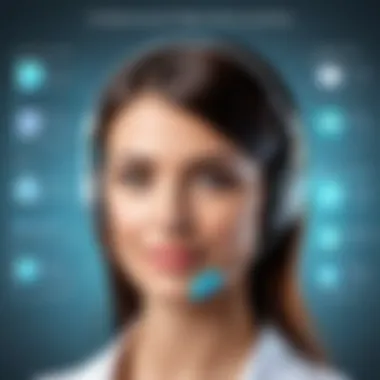
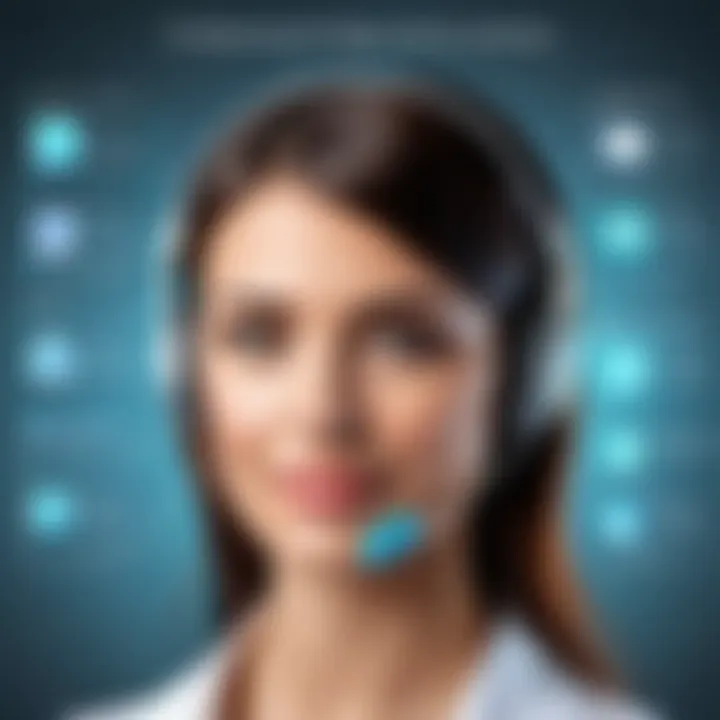
Challenges in Sentiment Analysis
Chat sentiment analysis, while offering a wealth of insights and benefits, is laden with challenges that can significantly impact its efficacy. Recognizing these hurdles is crucial for professionals aiming to derive meaningful conclusions from chat interactions. Without a clear understanding of these challenges, one might grapple with erroneous interpretations, which could lead to misguided business decisions or communication strategies. This section delves into the various complexities inherent in sentiment analysis, touching on the linguistic, cultural, and data-related factors that complicate the process.
Ambiguity in Language
Language, in all its intricacies and layers, poses a notable challenge in sentiment analysis. For instance, consider phrases like "I'm fine," which can be interpreted positively in one context and negatively in another, depending on tone, prior conversations, and cultural background. This ambiguity is not merely an academic concern; it reflects on how machine learning models interpret sentiments. If these models are trained without considering the context or nuances, they may misinterpret sarcasm, irony, or even hidden meanings.
"Understanding the subtleties of language is vital to accurately gauge sentiment."
There are idioms or regional slang that can further muddy the waters. A phrase that’s supportive in one culture might be considered dismissive in another. Therefore, the ability to decode this ambiguity necessitates sophisticated algorithms, capable of understanding context beyond simple keyword analysis.
Cultural Context Considerations
Cultural context is another layer that complicates sentiment analysis. Different cultures have varied expressions and interpretations of sentiment, making it critical to tailor sentiment analysis tools accordingly. For example, a straightforward expression of happiness in one culture could be received with skepticism in another. This divergence can skew results significantly if the analysis tools are not equipped to consider the user’s cultural background. The importance of understanding cultural nuances cannot be overstated, as an oversight here might result in branding failures or miscommunications.
More broadly, companies operating across borders need to take into account the expectations and language peculiarities of their global audience. Sometimes, humor or emotions are conveyed through nonverbal cues or specific jargon that detailed sentiment analysis tools might miss altogether. Hence, a one-size-fits-all approach can lead to serious errors in understanding.
Data Quality and Quantity Issues
Finally, the quality and quantity of data play pivotal roles in the effectiveness of sentiment analysis. High-quality, representative datasets are needed to train models adequately. If the data lacks diversity or contains biases, it directly affects the model's ability to generalize accurately to new inputs. Moreover, the volume of data sent through chats is immense, but sifting through this data for relevant insights becomes a monumental task. Poorly labeled or inconsistent data can lead the model astray, causing a ripple effect in the conclusions drawn from the sentiment analysis.
To mitigate these issues, organizations must invest time and resources into robust data collection processes. This involves not only gathering vast amounts of data but also ensuring it is accurate and labeled consistently. Ultimately, without overcoming the challenges posed by data quality and quantity, sentiment analysis can struggle to provide reliable insights.
Tools and Technologies for Sentiment Analysis
Navigating the landscape of chat sentiment analysis requires a robust understanding of the tools and technologies that power this fascinating field. The modern business environment thrives on data-driven insights, and sentiment analysis stands out as a crucial tool for unlocking the nuances behind customer conversations. With this understanding, organizations can better respond to customer needs, refine their marketing strategies, and enhance user experiences. Leveraging the right technologies isn’t just about implementation; it’s about capitalizing on the potential for growth and user satisfaction.
Popular Software Solutions
Several software solutions dominate the sentiment analysis market today, each with its unique strengths and features. Here’s a look at some of the leading tools:
- IBM Watson: This platform harnesses the power of AI to delve deep into natural language processing. Known for its reliability, Watson provides insights that assist businesses in understanding customer feedback effectively.
- Microsoft Text Analytics: Part of the Azure Cognitive Services, this tool offers a seamless integration with existing software, making it a favorite among IT professionals. Its ability to analyze mood changes over time is particularly beneficial for ongoing customer interactions.
- SAP Analytics Cloud: Beyond just sentiment analysis, this solution integrates data analytics with machine learning to provide a full suite of tools for data-driven decision-making.
Thanks to these solutions, businesses can process large volumes of data in mere moments, allowing for timely insights that keep pace with consumer sentiment.
APIs and Cloud-Based Services
APIs have revolutionized how businesses access and utilize sentiment analysis tools. With cloud-based services, organizations can scale solutions according to their requirements without heavy upfront investments. Here are some noteworthy APIs:
- Google Cloud Natural Language API: This API enables businesses to derive insights from text using advanced machine learning. Its user-friendly interface and scalability make it a preferred choice for many.
- AffectNet API: Focused on emotional responses, this service analyzes text input for various sentiment outputs. It provides a nuanced understanding of customer emotions, which is essential for reactionary marketing strategies.
- TextRazor: Known for its customizable and robust analytics, this API allows developers to fine-tune their sentiment analysis processes according to specific business needs.
The adaptability of cloud-based services means businesses can respond dynamically to changes in customer sentiment, keeping their strategies relevant.
Open-Source Libraries and Frameworks
For those with the ability to delve deeper into the development aspect, open-source libraries offer an incredible wealth of resources for building custom sentiment analysis tools. Popular choices include:
- NLTK (Natural Language Toolkit): A go-to library for many researchers, NLTK has extensive tools for text processing and sentiment classification, making it a staple for Python developers.
- spaCy: Known for its efficiency and speed, spaCy empowers developers to create robust pipelines for sentiment extraction, suitable for larger datasets.
- TensorFlow and Keras: While primarily known for deep learning, these frameworks can integrate powerful models for sentiment analysis, especially in dealing with complex datasets where traditional methods fall short.
Utilizing these libraries enables businesses to cultivate tailored solutions that align closely with their unique objectives, showcasing the flexibility and responsiveness of sentiment analysis technology.
Evaluating Sentiment Analysis Models
Evaluating sentiment analysis models is crucial for ensuring their effectiveness and relevance in real-world applications. Just like a well-oiled machine, a model must be fine-tuned to understand the nuances of sentiment in diverse contexts. This evaluation not only measures the model’s performance but also helps refine methodologies to enhance accuracy and practicability.
Performance Metrics Explained
When it comes to assessing sentiment analysis models, performance metrics play a pivotal role. These metrics are vital indicators of how well a model can discern sentiment from text. Some of the most commonly used performance metrics include:
- Accuracy: This tells you what portion of the total predictions were correct. While it’s straightforward, it doesn't always give the full picture, especially in imbalanced datasets.
- Precision and Recall:
- F1 Score: This is the harmonic mean of precision and recall, balancing the two metrics to give a more comprehensive view of the model’s performance.
- AUC-ROC: The Area Under the Receiver Operating Characteristics curve provides an aggregated measure of performance across all possible classification thresholds, informing on the trade-off between true positive rate and false positive rate.
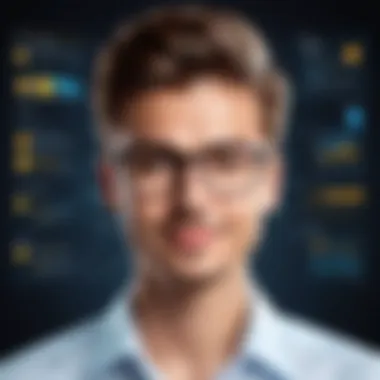
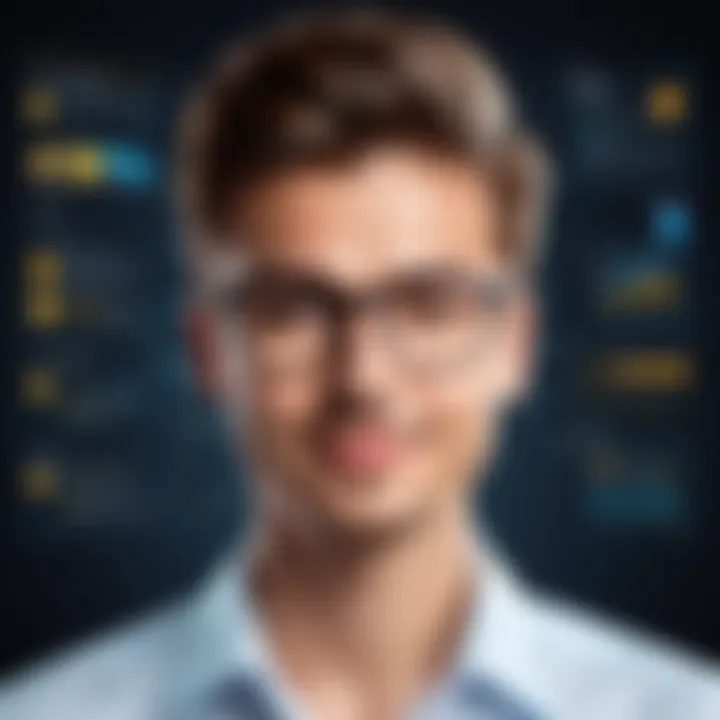
- Precision is the ratio of true positives to the sum of true positives and false positives. It reflects the model's ability to return only relevant instances.
- Recall, on the other hand, is the ratio of true positives to the sum of true positives and false negatives. It essentially gauges the model’s ability to identify all relevant instances.
These metrics together offer a multi-faceted view of the model's reliability, guiding necessary adjustments to improve overall performance.
Validation Techniques
Validation techniques serve as the backbone for determining how well a sentiment analysis model will function in a real-world scenario. They allow developers and data scientists to test the robustness and adaptability of their models. Common strategies for validation include:
- Cross-Validation: This approach divides the dataset into subsets, training the model multiple times on different subsets while validating it on the remaining data. It helps maintain a balance between training and testing, improving the model’s generalizability.
- Train-Test Split: Here, the data is divided into two parts. The model is trained on one set and tested on the other. This method is simple but effective in providing a quick estimate of model performance.
- K-Fold Validation: This technique involves dividing the data into k subsets and iteratively using each one as a validation set while training on the others. It’s particularly useful for smaller datasets and helps mitigate issues related to overfitting.
Using these techniques allows for rigorous evaluation, ensuring that a model is not just peaking at training accuracy but is genuinely capable of understanding sentiment in unseen data as well.
Case Studies of Successful Implementations
Examining real-world applications provides valuable insights into sentiment analysis models’ effectiveness and operationalization. Here are a couple of telling case studies that illustrate the impact of robust sentiment analysis:
Case Study: Starbucks
Starbucks employed sentiment analysis to enhance customer experience. By analyzing chat interactions, they identified frequent customer complaints about long wait times. Acting on this feedback enabled them to optimize staffing during peak hours. The result was a tangible increase in customer satisfaction metrics.
Case Study: Netflix
Netflix harnessed sentiment analysis to explore viewer reactions to original content. By analyzing comments and feedback across platforms, the company tailored its content strategy, resulting in a higher engagement rate. Their ability to gauge viewer sentiment accurately led to blockbuster series that resonated with audiences.
Through these case studies, it becomes evident that proper evaluation, along with effective implementation, can lead to substantial improvements in customer engagement and business performance. The journey of evaluating sentiment analysis models is not merely a technical exercise; it’s a strategic endeavor that can significantly shape how businesses interact with their customers.
Ethical Considerations in Sentiment Analysis
In the landscape of chat sentiment analysis, ethical considerations are crucial. As the technology evolves, so does the responsibility of ensuring that it is utilized in a manner that respects user rights while delivering accurate and actionable insights. The significance of ethics in this context isn't merely a footnote; rather, it sits at the very heart of how organizations approach data collection and analysis. Addressing ethical issues offers not just a safeguard against legal repercussions, but also enhances trust between businesses and their customers.
User Privacy and Data Security
When it comes to user privacy, sentiment analysis can tread a fine line. Extracting sentiments involves analyzing personal conversations, which can inadvertently invade individual privacy. Corporations must prioritize data protection protocols to ensure that user data is handled with care. Data should be anonymized or pseudonymized to prevent the identification of individuals.
Moreover, implementing strict security measures is a necessity. Unauthorized access to sensitive data can lead to breaches that harm both users and organizations. Companies should ensure compliance with data protection regulations such as the General Data Protection Regulation (GDPR). This involves not only securing data but also being transparent about how data is used and obtaining consent from users prior to analysis.
Bias in Sentiment Analysis Models
Bias presents another ethical hurdle in sentiment analysis. Models trained on skewed datasets can lead to unfair or inaccurate sentiment evaluations. For instance, linguistic nuances, dialects, and cultural contexts must be meticulously considered. If a model doesn't account for these variables, it might misinterpret sentiments, leading to misunderstandings that could impact customer satisfaction.
Tackling bias requires continuous monitoring and refining of the sentiment analysis models. It is not enough to simply rely on historical data; organizations need to stay alert to emerging trends in language and sentiment expression. This may involve incorporating feedback mechanisms that allow users to inform the model when a sentiment has been misjudged.
Regulatory Compliance Challenges
Regulatory compliance adds another layer of complexity. Different regions have diverse laws governing data usage, with varying requirements for transparency and user consent. Companies operating internationally face the challenge of navigating these regulations effectively. Non-compliance can lead to hefty fines and damage to reputation.
Understanding the regulatory landscape is critical for businesses that employ sentiment analysis. It requires staying updated with legal requirements in various jurisdictions, and aligning company protocols accordingly. This may involve creating comprehensive legal frameworks around data usage, including the implementation of features that allow users to opt in or out of data collection easily.
Future of Chat Sentiment Analysis
The future of chat sentiment analysis holds promise for transforming how organizations interact with customers and understand preferences. As we move further into a digital age where communication happens in rapid-fire chats and social media posts, the ability to grasp the sentiment behind these transactions becomes paramount. Not only does it enhance customer service but it also offers strategic insights that guide business decisions.
Emerging Trends and Innovations
Several trends are on the horizon for chat sentiment analysis. One significant development is the rise of contextual sentiment analysis, where algorithms understand the nuances of language, including sarcasm and cultural references. This means the tools will not just evaluate sentiment based on preset keywords but interpret phrases within their context. For instance, a message that reads, "Oh great, another update!" could be identified more accurately as sarcastic rather than simply positive, as traditional models might have done.
Another trend is the integration of multimodal data analysis. This involves analyzing not just text but also voice tonality and facial expressions during video calls. Such an approach offers a more holistic view of sentiment. For example, during a customer service interaction via video chat, body language could provide clues as to the customer's satisfaction level that is not captured through words alone.
Impact of AI Advancements
Artificial Intelligence continues to push the boundaries of what is possible in sentiment analysis. With advancements in natural language processing, AI can now analyze larger datasets at unprecedented speeds. This ability allows businesses to glean insights in real-time and respond more adeptly to customer needs. One of the byproducts of this AI evolution is the generation of sentiment prediction models, which forecast customer behavior based on historical data.
Moreover, AI's power to learn from mistakes helps improve sentiment analysis tools over time. As these systems become more sophisticated, they will increasingly use deep learning techniques to recognize patterns and trends in sentiment, making them indispensable in customer relationship management.
Integration with Other Technologies
As organizations adopt chat sentiment analysis, they will inevitably combine it with other technologies to optimize performance. For instance, integrating sentiment analysis with Customer Relationship Management (CRM) systems creates a feedback loop where customer insights feed directly into sales and marketing strategies. This synthesis allows for personalized marketing campaigns based on real customer sentiments, fostering deeper connections and increasing brand loyalty.
Chatbots, enhanced with sentiment analysis capabilities, will also become more prominent. These bots can gauge user emotions in conversations and adapt their responses accordingly. For example, a chatbot that detects frustration in a user's language may escalate an issue to a human agent proactively, improving customer satisfaction.
"The future of chat sentiment analysis is bright, as emerging trends and integration with other technologies will redefine customer interactions and business strategies."
Through these developments, businesses will not only adapt to changing communication dynamics but also leverage data to create strategies grounded in authentic customer sentiment. This evolution emphasizes that chat sentiment analysis will not just be a tool for today, but a cornerstone for successful digital communications in the future.